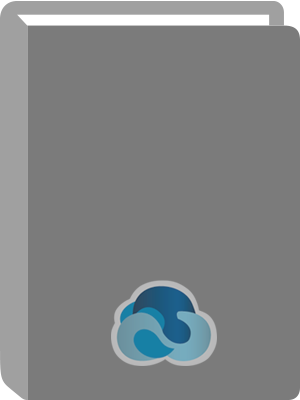
Available:*
Library | Item Barcode | Call Number | Material Type | Item Category 1 | Status |
---|---|---|---|---|---|
Searching... | 30000010275479 | QA76.9.D343 P66 2010 | Open Access Book | Book | Searching... |
On Order
Summary
Summary
Research in Natural Language Processing (NLP) has made tremendous progress in the last two decades by employing data-driven techniques. However, further major advances can be achieved by integrating linguistic, domain and world knowledge into statistical approaches. In this dissertation, a methodology is presented to extract this knowledge from Wikipedia, a resource which has attracted the attention of many researchers in the Artificial Intelligence (AI) community, mainly because it provides semi-structured information and a large amount of manual annotations. The proposed approach uses the category system found in Wikipedia as a conceptual network. Semantic relations between categories are labeled to produce a large-scale taxonomy. This resource is evaluated by comparing it with Cyc and WordNet, as well as through computing semantic similarity between words and using semantic similarity measures as features for a state-of-the-art co-reference resolution system. The results show that this taxonomy can be successfully deployed for NLP tasks and represents a valuable semantic resource for AI applications.
IOS Press is an international science, technical and medical publisher of high-quality books for academics, scientists, and professionals in all fields.
Some of the areas we publish in:
-Biomedicine
-Oncology
-Artificial intelligence
-Databases and information systems
-Maritime engineering
-Nanotechnology
-Geoengineering
-All aspects of physics
-E-governance
-E-commerce
-The knowledge economy
-Urban studies
-Arms control
-Understanding and responding to terrorism
-Medical informatics
-Computer Sciences