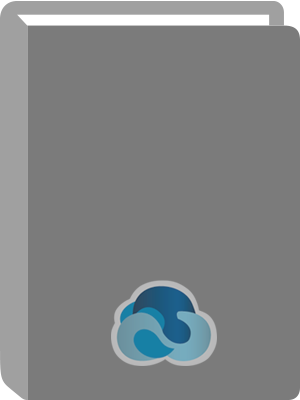
Available:*
Library | Item Barcode | Call Number | Material Type | Item Category 1 | Status |
---|---|---|---|---|---|
Searching... | 30000010186309 | QA280 P66 2009 | Open Access Book | Book | Searching... |
On Order
Summary
Summary
The problem of detecting abrupt changes in the behavior of an observed signal or time series arises in a variety of fields, including climate modeling, finance, image analysis, and security. Quickest detection refers to real-time detection of such changes as quickly as possible after they occur. Using the framework of optimal stopping theory, this book describes the fundamentals underpinning the field, providing the background necessary to design, analyze, and understand quickest detection algorithms. For the first time the authors bring together results which were previously scattered across disparate disciplines, and provide a unified treatment of several different approaches to the quickest detection problem. This book is essential reading for anyone who wants to understand the basic statistical procedures for change detection from a fundamental viewpoint, and for those interested in theoretical questions of change detection. It is ideal for graduate students and researchers of engineering, statistics, economics, and finance.
Author Notes
H. Vincent Poor is the Michael Henry Strater University Professor of Electrical Engineering, and Dean of the School Engineering and Applied Science, at Princeton University, from where he received his Ph.D. in 1977. Prior to joining the Princeton faculty in 1990, he was on the faculty of the University of Illinois at Urbana-Champaign, and has held visiting positions at a number of other institutions, including Imperial College, Harvard University, and Stanford University. He is a Fellow of the IEEE, the Institute of Mathematical Statistics, and the American Academy of Arts and Sciences, as well as being a member of the US National Academy of Engineering.
Olympia Hadjiliadis is an Assistant Professor in the Department of Mathematics at Brooklyn College of the City University of New York, where she is also a member of the graduate faculty of the Department of Computer Science. She was awarded her M.Math in Statistics and Finance in 1999 from the University of Waterloo, Canada. After receiving her Ph.D. in Statistics with distinction from Columbia University in 2005, Dr. Hadjiliadis joined the Electrical Engineering Department at Princeton as a Postdoctoral Fellow, where she was subsequently appointed as a Visiting Research Collaborator until 2008.
Table of Contents
List of figures | p. x |
Preface | p. xi |
Frequently used notation | p. xiii |
1 Introduction | p. 1 |
2 Probabilistic framework | p. 6 |
2.1 Introduction | p. 6 |
2.2 Basic setting | p. 6 |
2.2.1 Probability spaces | p. 6 |
2.2.2 Random variables | p. 7 |
2.2.3 Expectation | p. 8 |
2.2.4 Radon-Nikodym derivatives | p. 10 |
2.2.5 Conditional expectation and independence | p. 11 |
2.2.6 Random sequences | p. 15 |
2.3 Martingales and stopping times | p. 18 |
2.3.1 Martingales | p. 19 |
2.3.2 Stopping times | p. 24 |
2.3.3 Continuous-time analogs | p. 26 |
2.4 Brownian motion and Poisson processes | p. 27 |
2.4.1 Brownian motion | p. 28 |
2.4.2 Poisson processes | p. 30 |
2.5 Continuous-time semimartingales | p. 32 |
2.6 Stochastic integration | p. 34 |
3 Markov optimal stopping theory | p. 40 |
3.1 Introduction | p. 40 |
3.2 Markov optimal stopping problems | p. 40 |
3.3 The finite-horizon case: dynamic programming | p. 41 |
3.3.1 The general case | p. 41 |
3.3.2 The Markov case | p. 46 |
3.4 The infinite-horizon case | p. 50 |
3.4.1 A martingale interpretation of the finite-horizon results | p. 51 |
3.4.2 The infinite-horizon case for bounded reward | p. 52 |
3.4.3 The general infinite-horizon case | p. 55 |
3.4.4 The infinite-horizon case with Markov rewards | p. 59 |
3.5 Markov optimal stopping in continuous time | p. 60 |
3.6 Appendix: a proof of Lemma 3.8 | p. 61 |
4 Sequential detection | p. 65 |
4.1 Introduction | p. 65 |
4.2 Optimal detection | p. 65 |
4.3 Performance analysis | p. 74 |
4.4 The continuous-time case | p. 81 |
4.4.1 The Brownian case | p. 81 |
4.4.2 The Brownian case - an alternative proof | p. 86 |
4.4.3 An interesting extension of Wald-Wolfowitz | p. 90 |
4.4.4 The case of Itô processes | p. 91 |
4.4.5 The Poisson case | p. 93 |
4.4.6 The compound Poisson case | p. 100 |
4.5 Discussion | p. 101 |
5 Bayesian quickest detection | p. 102 |
5.1 Introduction | p. 102 |
5.2 Shiryaev's problem | p. 103 |
5.3 The continuous-time case | p. 109 |
5.3.1 Brownian observations | p. 109 |
5.3.2 Poisson observations | p. 115 |
5.4 A probability maximizing approach | p. 122 |
5.5 Other penalty functions | p. 124 |
5.6 A game theoretic formulation | p. 125 |
5.7 Discussion | p. 128 |
6 Non-Bayesian quickest detection | p. 130 |
6.1 Introduction | p. 130 |
6.2 Lorden's problem | p. 130 |
6.3 Performance of Page's test | p. 142 |
6.4 The continuous-time case | p. 144 |
6.4.1 Brownian observations | p. 144 |
6.4.2 It&ocaron; processes | p. 150 |
6.4.3 Brownian motion with an unknown drift parameter | p. 152 |
6.4.4 Poisson observations | p. 154 |
6.5 Asymptotic results | p. 157 |
6.5.1 Lorden's approach | p. 158 |
6.5.2 Brownian motion with two-sided alternatives | p. 167 |
6.6 Comments on the false-alarm constraint | p. 171 |
6.7 Discussion | p. 172 |
7 Additional topics | p. 174 |
7.1 Introduction | p. 174 |
7.2 Decentralized sequential and quickest detection | p. 175 |
7.2.1 Decentralized sequential detection with a fusion center | p. 176 |
7.2.2 Decentralized quickest detection with a fusion center | p. 184 |
7.2.3 Decentralized sequential detection without fusion | p. 189 |
7.3 Quickest detection with modeling uncertainty | p. 194 |
7.3.1 Robust quickest detection | p. 194 |
7.3.2 Adaptive quickest detection | p. 200 |
7.4 Quickest detection with dependent observations | p. 201 |
7.4.1 Quickest detection with independent likelihood ratio sequences | p. 201 |
7.4.2 Locally asymptotically normal distributions | p. 203 |
7.4.3 Sequential detection (local hypothesis approach) | p. 205 |
7.4.4 Quickest detection (local hypothesis approach) | p. 210 |
Bibliography | p. 213 |
Index | p. 225 |