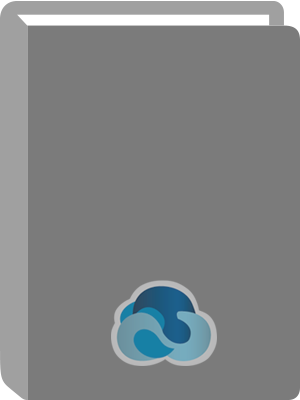
Available:*
Library | Item Barcode | Call Number | Material Type | Item Category 1 | Status |
---|---|---|---|---|---|
Searching... | 30000010107470 | CP 10335 | Computer File Accompanies Open Access Book | Compact Disc Accompanies Open Access Book | Searching... |
On Order
Summary
Summary
The past fifteen years has witnessed an explosive growth in the fundamental research and applications of artificial neural networks (ANNs) and fuzzy logic (FL). The main impetus behind this growth has been the ability of such methods to offer solutions not amenable to conventional techniques, particularly in application domains involving pattern recognition, prediction and control. Although the origins of ANNs and FL may be traced back to the 1940s and 1960s, respectively, the most rapid progress has only been achieved in the last fifteen years. This has been due to significant theoretical advances in our understanding of ANNs and FL, complemented by major technological developments in high-speed computing. In geophysics, ANNs and FL have enjoyed significant success and are now employed routinely in the following areas (amongst others): 1. Exploration Seismology. (a) Seismic data processing (trace editing; first break picking; deconvolution and multiple suppression; wavelet estimation; velocity analysis; noise identification/reduction; statics analysis; dataset matching/prediction, attenuation), (b) AVO analysis, (c) Chimneys, (d) Compression I dimensionality reduction, (e) Shear-wave analysis, (f) Interpretation (event tracking; lithology prediction and well-log analysis; prospect appraisal; hydrocarbon prediction; inversion; reservoir characterisation; quality assessment; tomography). 2. Earthquake Seismology and Subterranean Nuclear Explosions. 3. Mineral Exploration. 4. Electromagnetic I Potential Field Exploration. (a) Electromagnetic methods, (b) Potential field methods, (c) Ground penetrating radar, (d) Remote sensing, (e) inversion.
Table of Contents
List of Contributors | p. XI |
Preface | p. XIII |
Special Preface | p. XV |
Section A Exploration Seismology | |
1 A Review of Automated First-Break Picking and Seismic Trace Editing Techniques | p. 1 |
2 Automated Picking of Seismic First-Arrivals with Neural Networks | p. 13 |
3 Automated 3-D Horizon Tracking and Seismic Classification Using Artificial Neural Networks | p. 31 |
4 Seismic Horizon Picking Using a Hopfield Network | p. 45 |
5 Refinement of Deconvolution by Neural Networks | p. 57 |
6 Identification and Suppression of Multiple Reflections in Marine Seismic Data with Neural Networks | p. 71 |
7 Application of Artificial Neural Networks to Seismic Waveform Inversion | p. 89 |
8 Seismic Principal Components Analysis Using Neural Networks | p. 103 |
Section B Lithology, Well Logs, Prospectivity Mapping and Reservoir Characterisation | |
9 Fuzzy Classification for Lithology Determination From Well Logs | p. 125 |
10 Reservoir Property Estimation Using the Seismic Waveform and Feedforward Neural Networks | p. 143 |
11 An Information Integrated Approach for Reservoir Characterisation | p. 157 |
12 An Artificial Neural Network Method for Mineral Prospectivity Mapping: A Comparison with Fuzzy Logic and Bayesian Probability Methods | p. 179 |
13 Oil Reservoir Porosity Prediction Using a Neural Network Ensemble Approach | p. 197 |
14 Interpretation of Shallow Stratigraphic Facies Using a Self-Organizing Neural Network | p. 215 |
15 Neural Network Inversion of EM39 Induction Log Data | p. 231 |
Section C Electromagnetic Exploration | |
16 Interpretation of Airborne Electromagnetic Data with Neural Networks | p. 253 |
Section D Other Geophysical Applications | |
17 Integrated Processing and Imaging of Exploration Data: An Application of Fuzzy Logic | p. 269 |
18 Application of Multilayer Perceptrons to Earthquake Seismic Analysis | p. 287 |
Bibliography | p. 307 |