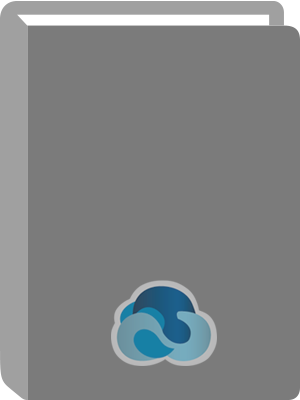
Available:*
Library | Item Barcode | Call Number | Material Type | Item Category 1 | Status |
---|---|---|---|---|---|
Searching... | 30000010128164 | TA330 A99 2006 | Open Access Book | Book | Searching... |
Searching... | 30000010215402 | TA330 A99 2006 | Open Access Book | Book | Searching... |
Searching... | 30000010215401 | TA330 A99 2006 | Open Access Book | Book | Searching... |
Searching... | 30000010270290 | TA330 A99 2006 | Open Access Book | Book | Searching... |
On Order
Summary
Summary
Engineers and scientists often need to solve complex problems with incomplete information resources, necessitating a proper treatment of uncertainty and a reliance on expert opinions. Uncertainty Modeling and Analysis in Engineering and the Sciences prepares current and future analysts and practitioners to understand the fundamentals of knowledge and ignorance, how to model and analyze uncertainty, and how to select appropriate analytical tools for particular problems.
This volume covers primary components of ignorance and their impact on practice and decision making. It provides an overview of the current state of uncertainty modeling and analysis, and reviews emerging theories while emphasizing practical applications in science and engineering.
The book introduces fundamental concepts of classical, fuzzy, and rough sets, probability, Bayesian methods, interval analysis, fuzzy arithmetic, interval probabilities, evidence theory, open-world models, sequences, and possibility theory. The authors present these methods to meet the needs of practitioners in many fields, emphasizing the practical use, limitations, advantages, and disadvantages of the methods.
Author Notes
Bilal M. Ayyub is a Professor of Civil and Environmental Engineering and the Director of the Center for Technology and Systems Management at the University of Maryland, College Park
George J. Klir is a Distinguished Professor of Systems Science at Binghamton University, State University of New York
Table of Contents
Chapter 1 Systems, Knowledge, and Ignorance | p. 1 |
1.1 Data Abundance and Uncertainty | p. 1 |
1.2 Systems Framework | p. 3 |
1.2.1 Systems Definitions and Modeling | p. 3 |
1.2.2 Realism and Constructivism in Systems Thinking | p. 5 |
1.2.3 Taxonomy of Systems | p. 6 |
1.2.3.1 Epistemological Categories of Systems | p. 7 |
1.2.3.2 Source (or Experimental Frame) Systems | p. 8 |
1.2.3.3 Data Systems | p. 11 |
1.2.3.4 Generative Systems | p. 11 |
1.2.3.5 Structure Systems | p. 13 |
1.2.3.6 Metasystems | p. 14 |
1.2.4 Disciplinary Roots of Systems Science | p. 25 |
1.2.5 Systems Knowledge, Methodology, and Metamethodology | p. 27 |
1.2.6 Complexity and Simplification of Systems | p. 29 |
1.2.7 Computational Complexity and Limitations | p. 32 |
1.3 Knowledge | p. 37 |
1.3.1 Terminology and Definitions | p. 37 |
1.3.2 Absolute Reality and Absolute Knowledge | p. 39 |
1.3.3 Knowledge, Information, and Opinions | p. 40 |
1.3.4 Reasoning, Science, and Uncertainty | p. 43 |
1.3.5 Cognition and Cognitive Science | p. 46 |
1.4 Ignorance | p. 48 |
1.4.1 Knowledge and Ignorance | p. 48 |
1.4.2 Ignorance Classification and Hierarchy | p. 50 |
1.4.2.1 Ignorance Classification | p. 50 |
1.4.2.2 Ignorance Hierarchy | p. 52 |
1.4.3 Uncertainty Theories and Classifications | p. 56 |
1.4.3.1 Ignorance Types, Mathematical Theories, and Applications | p. 56 |
1.4.3.2 Aleatory and Epistemic Uncertainties | p. 56 |
1.4.3.3 Uncertainty in System Abstraction | p. 59 |
1.5 From Data to Knowledge for Decision Making | p. 63 |
Exercise Problems | p. 65 |
Chapter 2 Encoding Data and Expressing Information | p. 67 |
2.1 Introduction | p. 67 |
2.2 Identification and Classification of Theories | p. 67 |
2.3 Crisp Sets and Operations | p. 70 |
2.3.1 A Universe and Its Elements | p. 70 |
2.3.2 Classical (Crisp) Sets and Events | p. 71 |
2.3.3 Properties of Sets and Subsets | p. 72 |
2.3.4 Characteristic Function | p. 74 |
2.3.5 Sample Space and Events | p. 74 |
2.3.6 Euclidean Vector Space and Set Convexity | p. 75 |
2.3.7 Venn-Euler Diagrams | p. 75 |
2.3.8 Basic Operations on Sets | p. 76 |
2.3.9 Power Sets | p. 78 |
2.4 Fuzzy Sets and Operations | p. 79 |
2.4.1 Membership Function | p. 80 |
2.4.2 [alpha]-Cut Representation of Sets | p. 84 |
2.4.3 Fuzzy Venn-Euler Diagrams | p. 85 |
2.4.4 Operations on Fuzzy Sets | p. 85 |
2.4.5 Cardinality of Fuzzy Sets | p. 97 |
2.4.6 Fuzzy Subsets | p. 98 |
2.4.7 Fuzzy Intervals, Numbers, and Arithmetic | p. 99 |
2.4.8 Fuzzy Relations | p. 107 |
2.4.9 Fuzzified and Fuzzy Functions | p. 112 |
2.5 Generalized Measures | p. 114 |
2.6 Rough Sets and Operations | p. 115 |
2.6.1 Rough Set Definitions | p. 115 |
2.6.2 Rough Set Operations | p. 117 |
2.6.3 Membership Functions for Rough Sets | p. 118 |
2.6.4 Rough Functions | p. 119 |
2.7 Gray Systems and Operations | p. 121 |
Exercise Problems | p. 121 |
Chapter 3 Uncertainty and Information Synthesis | p. 127 |
3.1 Synthesis for a Goal | p. 127 |
3.2 Knowledge, Systems, Uncertainty, and Information | p. 127 |
3.3 Measure Theory and Classical Measures | p. 129 |
3.4 Monotone Measures and Their Classification | p. 131 |
3.4.1 Definition of Monotone Measures | p. 131 |
3.4.2 Classifying Monotone Measures | p. 132 |
3.5 Dempster-Shafer Evidence Theory | p. 136 |
3.5.1 Belief Measures | p. 136 |
3.5.2 Plausibility Measure | p. 137 |
3.5.3 Interpretation of Belief and Plausibility Measures | p. 137 |
3.5.4 Mobius Representation as a Basic Assignment | p. 138 |
3.5.5 Combination of Evidence | p. 139 |
3.5.5.1 Dempster's Rule of Combination | p. 139 |
3.5.5.2 Yager's Rule of Combination | p. 140 |
3.5.5.3 Inagaki's Rule of Combination | p. 140 |
3.5.5.4 Mixed or Averaging Rule of Combination | p. 141 |
3.6 Possibility Theory | p. 147 |
3.6.1 Classical Possibility Theory | p. 147 |
3.6.2 Theory of Graded Possibilities | p. 148 |
3.7 Probability Theory | p. 151 |
3.7.1 Relationship between Evidence Theory and Probability Theory | p. 151 |
3.7.2 Classical Definitions of Probability | p. 151 |
3.7.3 Linguistic Probabilities | p. 153 |
3.7.4 Failure Rates | p. 153 |
3.7.5 Central Tendency Measures | p. 155 |
3.7.6 Dispersion (or Variability) | p. 156 |
3.7.7 Percentile Values | p. 157 |
3.7.8 Statistical Uncertainty | p. 158 |
3.7.9 Bayesian Probabilities | p. 160 |
3.8 Imprecise Probabilities | p. 166 |
3.8.1 Interval Probabilities | p. 167 |
3.8.2 Interval Cumulative Distribution Functions | p. 171 |
3.8.3 Dependence Modeling and Measures | p. 174 |
3.8.3.1 Perfect Independence | p. 175 |
3.8.3.2 Mutual Exclusion | p. 176 |
3.8.4 Opposite Dependence | p. 177 |
3.8.5 Perfect Dependence | p. 177 |
3.8.6 Partial Dependence | p. 178 |
3.8.7 Correlation between Random Variables | p. 178 |
3.8.7.1 Correlation Based on Probability Theory | p. 178 |
3.8.7.2 Statistical Correlation | p. 182 |
3.8.8 Correlation between Events | p. 184 |
3.8.9 Unknown Dependence between Events | p. 185 |
3.8.10 Unknown Positive Dependence between Events | p. 186 |
3.8.11 Unknown Negative Dependence between Events | p. 186 |
3.8.12 Probability Bounds | p. 186 |
3.9 Fuzzy Measures and Fuzzy Integrals | p. 193 |
Exercise Problems | p. 197 |
Chapter 4 Uncertainty Measures | p. 203 |
4.1 Introduction | p. 203 |
4.2 Uncertainty Measures: Definition and Types | p. 203 |
4.3 Nonspecificity Measures | p. 205 |
4.3.1 Hartley Measure | p. 205 |
4.3.2 Hartley-Like Measure | p. 210 |
4.3.3 Evidence Nonspecificity | p. 211 |
4.3.4 Nonspecificity of Graded Possibility | p. 212 |
4.3.5 Nonspecificity of Fuzzy Sets or U-Uncertainty | p. 213 |
4.4 Entropy-Like Measures | p. 215 |
4.4.1 Shannon Entropy for Probability Distributions | p. 216 |
4.4.2 Discrepancy Measure | p. 219 |
4.4.3 Entropy Measures for Evidence Theory | p. 219 |
4.4.3.1 Measure of Dissonance | p. 219 |
4.4.3.2 Measure of Confusion | p. 220 |
4.4.4 Aggregate and Disaggregate Uncertainty in Evidence Theory | p. 221 |
4.5 Fuzziness Measure | p. 224 |
4.6 Application: Combining Expert Opinions | p. 226 |
4.6.1 Consensus Combination of Opinions | p. 226 |
4.6.2 Percentiles for Combining Opinions | p. 226 |
4.6.3 Weighted Combinations of Opinions | p. 227 |
Exercise Problems | p. 230 |
Chapter 5 Uncertainty-Based Principles and Knowledge Construction | p. 233 |
5.1 Introduction | p. 233 |
5.2 Construction of Knowledge | p. 234 |
5.3 Minimum Uncertainty Principle | p. 235 |
5.4 Maximum Uncertainty Principle | p. 236 |
5.5 Uncertainty Invariance Principle | p. 246 |
5.6 Methods for Open-World Analysis | p. 248 |
5.6.1 Statistical Estimators for Sequences and Patterns | p. 249 |
5.6.1.1 Laplace Model | p. 249 |
5.6.1.2 Add-c Model | p. 250 |
5.6.1.3 Witten-Bell Model | p. 251 |
5.6.2 Transferable Belief Model | p. 252 |
5.6.3 Open-World Assumption Mathematical Framework | p. 254 |
5.6.4 Evidential Reasoning Mechanism, Belief Revision, and Diagnostics | p. 255 |
Exercise Problems | p. 256 |
Chapter 6 Uncertainty Propagation for Systems | p. 259 |
6.1 Introduction | p. 259 |
6.2 Fundamental Methods for Propagating Uncertainty | p. 260 |
6.2.1 Analytic Probabilistic Methods | p. 260 |
6.2.1.1 Probability Distributions for Dependent Random Variables | p. 261 |
6.2.1.2 Mathematical Expectations | p. 265 |
6.2.1.3 Approximate Methods | p. 266 |
6.2.2 Simulation Methods | p. 268 |
6.2.3 Vertex Method for Functions of Fuzzy Variables | p. 271 |
6.3 Propagation of Mixed Uncertainty Types | p. 275 |
6.3.1 A Fundamental Input-Output System | p. 275 |
6.3.2 Interval Parameters | p. 276 |
6.3.3 A Power as an Interval and a Set of Intervals | p. 277 |
6.3.3.1 A Consonant or Nested Set of Intervals | p. 278 |
6.3.3.2 A Consistent Set of Intervals | p. 280 |
6.3.3.3 An Arbitrary Set of Intervals | p. 282 |
6.3.4 Sets of Intervals | p. 284 |
6.3.4.1 Consonant or Nested Sets of Intervals | p. 284 |
6.3.4.2 Consistent Sets of Intervals | p. 286 |
6.3.4.3 Arbitrary Sets of Intervals | p. 288 |
6.3.5 A Power as an Interval and as a Set of Intervals | p. 288 |
6.3.5.1 Power Intervals and Lognormally Distributed Parameter | p. 288 |
6.3.5.2 A Power as an Interval and an Uncertain Lognormally Distributed Parameter | p. 290 |
6.3.5.3 A Set of Power Intervals and an Uncertain Lognormally Distributed Parameter | p. 290 |
Exercise Problems | p. 291 |
Chapter 7 Expert Opinions and Elicitation Methods | p. 297 |
7.1 Introduction | p. 297 |
7.2 Terminology | p. 298 |
7.3 Classification of Issues, Study Levels, Experts, and Process Outcomes | p. 298 |
7.4 Process Definition | p. 302 |
7.5 Need Identification for Expert Opinion Elicitation | p. 302 |
7.6 Selection of Study Level and Study Leader | p. 303 |
7.7 Selection of Peer Reviewers and Experts | p. 304 |
7.7.1 Selection of Peer Reviewers | p. 304 |
7.7.2 Identification and Selection of Experts | p. 304 |
7.7.3 Items Needed by Experts and Reviewers before the Expert Opinion Elicitation Meeting | p. 306 |
7.8 Identification, Selection, and Development of Technical Issues | p. 307 |
7.9 Elicitation of Opinions | p. 308 |
7.9.1 Issue Familiarization of Experts | p. 308 |
7.9.2 Training of Experts | p. 308 |
7.9.3 Elicitation and Collection of Opinions | p. 309 |
7.9.4 Aggregation and Presentation of Results | p. 309 |
7.9.5 Group Interaction, Discussion, and Revision by Experts | p. 310 |
7.10 Documentation and Communication | p. 310 |
Exercise Problems | p. 318 |
Chapter 8 Visualization of Uncertainty | p. 321 |
8.1 Introduction | p. 321 |
8.2 Visualization Methods | p. 324 |
8.2.1 Statistical and Probability-Based Visualization | p. 324 |
8.2.2 Point and Global Visualization | p. 324 |
8.2.3 Use of Colors | p. 326 |
8.2.4 Financial Visualization | p. 327 |
8.2.5 Icons, Ontology, and Lexicon | p. 328 |
8.2.5.1 Emoticons | p. 328 |
8.2.5.2 Ignoricons and Uncerticons | p. 328 |
8.3 Criteria and Metrics for Assessing Visualization Methods | p. 330 |
8.3.1 Definition of Primary Selection Criteria and Weight Factors | p. 330 |
8.3.2 Definition and Selection Subcriteria and Weight Factors | p. 333 |
8.3.3 Summary of Criteria, Subcriteria, and Weight Factors | p. 335 |
8.3.4 Design of Visualization Method | p. 335 |
8.3.5 Assessment Methods and Experimental Protocol | p. 337 |
8.3.6 Candidate Shapes for Ignoricons and Uncerticons | p. 339 |
8.4 Intelligent Agents for Icon Selection, Display, and Updating | p. 340 |
8.4.1 Intelligent Agents | p. 340 |
8.4.2 Information Uncertainty Agent | p. 341 |
8.4.3 Processing Information for Symbology Selection | p. 342 |
8.5 Ignorance Markup Language | p. 343 |
Exercise Problems | p. 343 |
Appendix A Historical Perspectives on Knowledge | p. 345 |
Bibliography | p. 353 |
Index | p. 369 |