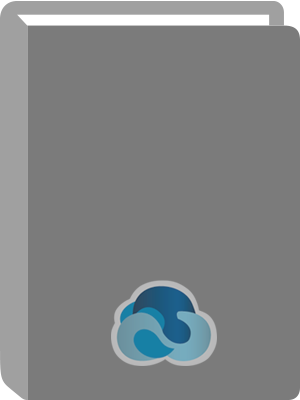
Title:
Introduction to modern time series analysis
Personal Author:
Publication Information:
Berlin : Springer, 2007
Physical Description:
ix, 274 p. : ill. ; 24 cm.
ISBN:
9783540732907
9783540732914
General Note:
Also available in online version
Subject Term:
Added Author:
Electronic Access:
Fulltext
DSP_RESTRICTION_NOTE:
Remote access restricted to users with a valid UTM ID via VPN
Available:*
Library | Item Barcode | Call Number | Material Type | Item Category 1 | Status |
---|---|---|---|---|---|
Searching... | 30000010178167 | QA280 K57 2007 | Open Access Book | Book | Searching... |
On Order
Summary
Summary
This book contains the most important approaches to analyze time series which may be stationary or nonstationary. It starts with modeling and forecasting univariate time series and then presents Granger causality tests and vector autoregressive models for multiple stationary time series. It also covers modeling volatilities of financial time series with autoregressive conditional heteroskedastic models.
Table of Contents
Preface | p. V |
1 Introduction and Basics | p. 1 |
1.1 The Historical Development of Time Series Analysis | p. 2 |
1.2 Graphical Representations of Economic Time Series | p. 5 |
1.3 Ergodicity and Stationarity | p. 12 |
1.4 The Wold Decomposition | p. 21 |
References | p. 22 |
2 Univariate Stationary Processes | p. 27 |
2.1 Autoregressive Processes | p. 27 |
2.1.1 First Order Autoregressive Processes | p. 27 |
2.1.2 Second Order Autoregressive Processes | p. 40 |
2.1.3 Higher Order Autoregressive Processes | p. 49 |
2.1.4 The Partial Autocorrelation Function | p. 52 |
2.1.5 Estimating Autoregressive Processes | p. 56 |
2.2 Moving Average Processes | p. 57 |
2.2.1 First Order Moving Average Processes | p. 58 |
2.2.2 Higher Order Moving Average Processes | p. 64 |
2.3 Mixed Processes | p. 67 |
2.3.1 ARMA(1,1) Processes | p. 67 |
2.3.2 ARMA(p,q) Processes | p. 73 |
2.4 Forecasting | p. 75 |
2.4.1 Forecasts with Minimal Mean Squared Errors | p. 75 |
2.4.2 Forecasts of ARMA(p,q) Processes | p. 80 |
2.4.3 Evaluation of Forecasts | p. 84 |
2.5 The Relation between Econometric Models and ARMA Processes | p. 87 |
References | p. 88 |
3 Granger Causality | p. 93 |
3.1 The Definition of Granger Causality | p. 95 |
3.2 Characterisations of Causal Relations in Bivariate Models | p. 97 |
3.2.1 Characterisations of Causal Relations using the Autoregressive and Moving Average Representations | p. 97 |
3.2.2 Characterising Causal Relations by Using the Residuals of the Univariate Processes | p. 99 |
3.3 Causality Tests | p. 102 |
3.3.1 The Direct Granger Procedure | p. 102 |
3.3.2 The Haugh-Pierce Test | p. 106 |
3.3.3 The Hsiao Procedure | p. 110 |
3.4 Applying Causality Tests in a Multivariate Setting | p. 114 |
3.4.1 The Direct Granger Procedure with More Than Two Variables | p. 114 |
3.4.2 Interpreting the Results of Bivariate Tests in Systems With More Than Two Variables | p. 117 |
3.5 Concluding Remarks | p. 118 |
References | p. 120 |
4 Vector Autoregressive Processes | p. 125 |
4.1 Representation of the System | p. 127 |
4.2 Granger Causality | p. 136 |
4.3 Impulse Response Analysis | p. 138 |
4.4 Variance Decomposition | p. 144 |
4.5 Concluding Remarks | p. 149 |
References | p. 150 |
5 Nonstationary Processes | p. 153 |
5.1 Forms of Nonstationarity | p. 153 |
5.2 Trend Elimination | p. 159 |
5.3 Unit Root Tests | p. 163 |
5.3.1 Dickey-Fuller Tests | p. 165 |
5.3.2 The Phillips-Perron Test | p. 171 |
5.3.3 Unit Root Tests and Structural Breaks | p. 176 |
5.3.4 A Test with the Null Hypothesis of Stationarity | p. 178 |
5.4 Decomposition of Time Series | p. 180 |
5.5 Further Developments | p. 187 |
5.5.1 Fractional Integration | p. 187 |
5.5.2 Seasonal Integration | p. 189 |
5.6 Deterministic versus Stochastic Trends in Economic Time Series | p. 191 |
References | p. 194 |
6 Cointegration | p. 199 |
6.1 Definition and Properties of Cointegrated Processes | p. 203 |
6.2 Cointegration in Single Equation Models: Representation,Estimation and Testing | p. 205 |
6.2.1 Bivariate Cointegration | p. 205 |
6.2.2 Cointegration with More Than Two Variables | p. 208 |
6.2.3 Testing Cointegration in Static Models | p. 209 |
6.2.4 Testing Cointegration in Dynamic Models | p. 213 |
6.3 Cointegration in Vector Autoregressive Models | p. 218 |
6.3.1 The Vector Error Correction Representation | p. 219 |
6.3.2 The Johansen Approach | p. 222 |
6.3.3 Analysis of Vector Error Correction Models | p. 229 |
6.4 Cointegration and Economic Theory | p. 234 |
References | p. 235 |
7 Autoregressive Conditional Heteroskedasticity | p. 241 |
7.1 ARCH Models | p. 245 |
7.1.1 Definition and Representation | p. 245 |
7.1.2 Unconditional Moments | p. 248 |
7.1.3 Temporal Aggregation | p. 249 |
7.2 Generalised ARCH Models | p. 252 |
7.2.1 GARCH Models | p. 252 |
7.2.2 The GARCH(1,1) process | p. 254 |
7.2.3 Nonlinear Extensions | p. 257 |
7.3 Estimation and Testing | p. 259 |
7.4 ARCH/GARCH Models as Instruments of Financial Market Analysis | p. 261 |
References | p. 263 |
Index of Names and Authors | p. 267 |
Subject Index | p. 271 |