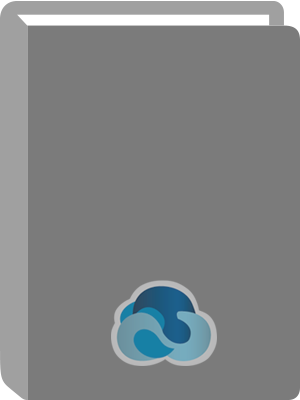
Available:*
Library | Item Barcode | Call Number | Material Type | Item Category 1 | Status |
---|---|---|---|---|---|
Searching... | 30000010199996 | QA279.5 G54 2008 | Open Access Book | Book | Searching... |
On Order
Summary
Summary
The first edition of Bayesian Methods: A Social and Behavioral Sciences Approach helped pave the way for Bayesian approaches to become more prominent in social science methodology. While the focus remains on practical modeling and basic theory as well as on intuitive explanations and derivations without skipping steps, this second edition incorporates the latest methodology and recent changes in software offerings.
New to the Second Edition
Two chapters on Markov chain Monte Carlo (MCMC) that cover ergodicity, convergence, mixing, simulated annealing, reversible jump MCMC, and coupling
Expanded coverage of Bayesian linear and hierarchical models
More technical and philosophical details on prior distributions
A dedicated R package (BaM) with data and code for the examples as well as a set of functions for practical purposes such as calculating highest posterior density (HPD) intervals
Requiring only a basic working knowledge of linear algebra and calculus, this text is one of the few to offer a graduate-level introduction to Bayesian statistics for social scientists. It first introduces Bayesian statistics and inference, before moving on to assess model quality and fit. Subsequent chapters examine hierarchical models within a Bayesian context and explore MCMC techniques and other numerical methods. Concentrating on practical computing issues, the author includes specific details for Bayesian model building and testing and uses the R and BUGS software for examples and exercises.
Table of Contents
Background and Introduction to Bayesian Methods |
Likelihood Interference and the Generalized Linear Model |
Bayesian Models for Explaining Single Variables |
Robustness and Sensitivity |
Assessing Model Quality |
The Other MC: Markov Chain Theory |
Putting MC and MC Together: Markov Chain Monte Carlo |
Numerical Issues in MCMC |
Recent Developments in MCMC Theory |
Bayesian Hierarchal Models and Empirical Bayes |
Bayesian Time Series and Survival Models |