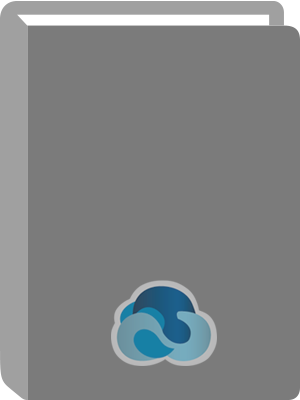
Available:*
Library | Item Barcode | Call Number | Material Type | Item Category 1 | Status |
---|---|---|---|---|---|
Searching... | 30000010058898 | TS156 T334 2002 | Open Access Book | Book | Searching... |
On Order
Summary
Summary
Cutting-edge measurement technology for multidimensional systems
The Mahalanobis-Taguchi Strategy presents methods for developing multidimensional measurement scales that are up to date with the most current trends in multivariate diagnosis/pattern recognition-namely, using measures and procedures that are data analytic and not dependent upon the distribution of the characteristics defining the system. Applications for these measurement scales are also explored across a wide range of disciplines from manufacturing to medicine.
This book presents methods that integrate mathematical and statistical concepts such as Mahalanobis distance and Gram-Schmidt's orthogonalization method with the principles of Taguchi methods. These completely new systems of measurement and analysis move beyond anything Dr. Taguchi has done in the past. Coverage includes the refined Mahalanobis-Taguchi system, the Mahalanobis-Taguchi-Gram-Schmidt method, the Adjoint Matrix method, and other advanced topics, along with a detailed examination of each method. In addition to examining how real-world problems are solved using these methods, critical comparisons are made between the methods covered here and existing multivariate diagnosis/pattern recognition techniques.
The Mahalanobis-Taguchi Strategy: A Pattern Technology System is an essential book for engineers, designers, and statistical quality experts and programmers in the fields of engineering and computer science, as well as researchers in finance, medicine, statistics, and general science.
Author Notes
GENICHI TAGUCHI, DSc, is Executive Director of the American Supplier Institute and President of Ohken Associates in Japan. Dr. Taguchi, an international authority in quality engineering and robust design, has been awarded the prestigious Deming Prize in 1960 and Willard F. Rockwell Medal in 1986. He was the recipient of the 1995 ASQ's Shewhart medal. In 1998, Dr. Taguchi was elected as an Honorary Member of the ASQ, the ASME, and the Honorary Chairman of the Japanese Quality Engineering Society. He was inducted into the World Level of the Hall of Fame for Engineering, Science, and Technology, USA, in 1998, and the Automotive Hall of Fame, USA, in 1997. In 2000, he was recognized as the Quality Champion of the twentieth century. He authored or coauthored more than 400 technical articles in leading journals and forty books.
RAJESH JUGULUM, Ph.D. is a researcher in the Department of Mechanical Engineering of Massachusetts Institute of Technology. In 2000, he completed his Ph.D. under the guidance of Dr. Genichi Taguchi in Wayne State University. In 1999, he shared ASQ research fellowship grant and in 2000 he received ASQ's Richard A. Freund international scholarship. He was featured as "Face of Quality" in September 2001 issue of Quality Progress. He is the recipient of 2002 ASQ's Feigenbaum medal. He has authored or co-authored several articles in leading technical journals. He is a member of the ASQ and the Japanese Quality Engineering Society and a Fellow of the Royal Statistical Society.
Table of Contents
Preface |
Acknowledgments |
Terms and Symbols |
Definitions of Mathematical and Statistical Terms |
1 Introduction |
1.1 The Goal |
1.2 The Nature of a Multidimensional System |
1.2.1 Description of Multidimensional Systems |
1.2.2 Correlations between the Variables |
1.2.3 Mahalanobis Distance |
1.2.4 Robust Engineering/ Taguchi Methods |
1.3 Multivariate DiagnosisThe State of the Art |
1.3.1 Principal Component Analysis |
1.3.2 Discrimination and Classification Method |
1.3.3 Stepwise Regression |
1.3.4 Test of Additional Information (Raos Test) |
1.3.5 Multiple Regression |
1.3.6 Multivariate Process Control Charts |
1.3.7 Artificial Neural Networks |
1.4 Approach |
1.4.1 Classification versus Measurement |
1.4.2 Normals versus Abnormals |
1.4.3 Probabilistic versus Data Analytic |
1.4.4 Dimensionality Reduction |
1.5 Refining the Solution Strategy |
1.6 Guide to This Book |
2 MTS and MTGS |
2.1 A Discussion of Mahalanobis Distance |
2.2 Objectives of MTS and MTGS |
2.2.1 Mahalanobis Distance (Inverse MatrixMethod) |
2.2.2 Gram-Schmidt Orthogonalization Process |
2.2.3 Proof That Equations 2.2 and 2.3 Are theSame |
2.2.4 Calculation of the Mean of the MahalanobisSpace |
2.3 Steps in MTS |
2.4 Steps in MTGS |
2.5 Discussion of Medical Diagnosis Data: Use of MTGS andMTS Methods |
2.6 Conclusions |
3 Advantages and Limitations of MTS and MTGS |
3.1 Direction of Abnormalities |
3.1.1 The Gram-Schmidt Process |
3.1.2 Identification of the Direction of Abnormals |
3.1.3 Decision Rule for Higher Dimensions |
3.2 Example of a Graduate Admission System |
3.3 Multicollinearity |
3.4 A Discussion of Partial Correlations |
3.5 Conclusions |
4 Role of Orthogonal Arrays and Signal-to-Noise Ratios inMultivariate Diagnosis |
4.1 Role of Orthogonal Arrays |
4.2 Role of S/ N Ratios |
4.3 Advantages of S/ N ratios |
4.3.1 S/ N Ratio as a Simple Measure to Identify UsefulVariables |
4.3.2 S/ N Ratio as a Measure of Functionality of theSystem |
4.3.3 S/ N Ratio to Predict the Given Conditions |
4.4 Conclusions |
5 Treatment of Categorical Data in MTS/MTGS Methods |
5.1 MTS/ MTGS with Categorical Data |
5.2 A Sales and Marketing Application |
5.2.1 Selection of Suitable Variables |
5.2.2 Description of the Variables |
5.2.3 Construction of Mahalanobis Space |
5.2.4 Validation of the Measurement Scale |
5.2.5 Identification of Useful Variables (DevelopingStage) |
5.2.6 S/ N Ratio of the System (Before and After) |
5.3 Conclusions |
6 MTS/ MTGS under a Noise Environment |
6.1 MTS/ MTGS with Noise Factors |
6.1.1 Treat Each Level of the Noise FactorSeparately |
6.1.2 Include the Noise Factor as One of theVariables |
6.1.3 Combine Variables of Different Levels of theNoise Factor |
6.1.4 Do Not Consider the Noise Factor If It Cannot BeMeasured |
6.2 Conclusions |
7 Determination of ThresholdsA Loss Function Approach |
7.1 Why Threshold Is Required in MTS/ MTGS |
7.2 Quadratic Loss Function |
7.2.1 QLF for the Nominal-the-Best Characteristic |
7.2.2 QLF for the Larger-the-Better Characteristic |
7.2.3 QLF for the Smaller-the-BetterCharacteristic |
7.3 QLF for MTS/ MTGS |
7.3.1 Determination of Threshold |
7.3.2 When Only Good Abnormals Are Present |
7.4 Examples |
7.4.1 Medical Diagnosis Case |
7.4.2 A Student Admission System |
7.5 Conclusions |
8 Standard Error of the Measurement Scale |
8.1 Why Mahalanobis Distance Is Used for Constructing theMeasurement Scale |
8.2 Standard Error of the Measurement Scale |
8.3 Standard Error for the Medical Diagnosis Example |
8.4 Conclusions |
9 Advance Topics in Multivariate Diagnosis |
9.1 Multivariate Diagnosis Using the Adjoint MatrixMethod |
9.1.1 Related Topics of Matrix Theory |
9.1.2 Adjoint Matrix Method f |