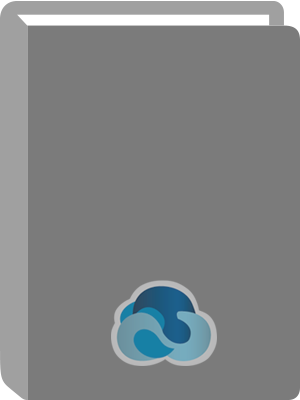
Available:*
Library | Item Barcode | Call Number | Material Type | Item Category 1 | Status |
---|---|---|---|---|---|
Searching... | 30000003155870 | QA76.5 J82 1990 | Open Access Book | Book | Searching... |
On Order
Summary
Summary
Using the tools of complexity theory, Stephen Judd develops a formal description of associative learning in connectionist networks. He rigorously exposes the computational difficulties in training neural networks and explores how certain design principles will or will not make the problems easier.Judd looks beyond the scope of any one particular learning rule, at a level above the details of neurons. There he finds new issues that arise when great numbers of neurons are employed and he offers fresh insights into design principles that could guide the construction of artificial and biological neural networks.The first part of the book describes the motivations and goals of the study and relates them to current scientific theory. It provides an overview of the major ideas, formulates the general learning problem with an eye to the computational complexity of the task, reviews current theory on learning, relates the book's model of learning to other models outside the connectionist paradigm, and sets out to examine scale-up issues in connectionist learning.Later chapters prove the intractability of the general case of memorizing in networks, elaborate on implications of this intractability and point out several corollaries applying to various special subcases. Judd refines the distinctive characteristics of the difficulties with families of shallow networks, addresses concerns about the ability of neural networks to generalize, and summarizes the results, implications, and possible extensions of the work.
Neural Network Design and the Complexity of Learning is included in the Network Modeling and Connectionism series edited by Jeffrey Elman.
Reviews 1
Choice Review
Motivated by a desire to model brain function, information specialists currently have wide interest in understanding so-called connectionist models of computation, models in which large numbers of processors or nodes, each performing only simple tasks, are, like neurons, highly interconnected. This book focuses on the study of learning in such networks. Judd attempts to explain why empirical successes have been limited to very small networks. His basic tool, the notion of intractability, in the form of NP-completeness, is clearly set forth. He abstracts from the learning process a very precise problem, called the loading problem, which is basically to pick a function for each node in a predetermined network so that the network will successfully perform a certain memory task. The main result is that the loading problem is almost surely intractable, though some constrained versions of it are easy. The results are quite strong and general; they will be relevant to the formulation of more refined connectionist models of learning. Judd deftly writes for two audiences by isolating the mathematical technicalities from more general considerations. The lively interaction of artificial intelligence and complexity theory make this book quite unusual. Advanced undergraduates and up. -D. V. Feldman, University of New Hampshire