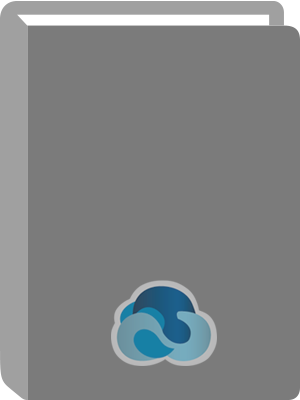
Title:
New directions in bioprocess modeling and control : maximizing process analytical technology benefits
Personal Author:
Publication Information:
Research Triangle Park, NC : ISA, 2007
Physical Description:
xii, 338 p. : ill. ; 26 cm.
ISBN:
9781556179051
Subject Term:
Added Author:
Available:*
Library | Item Barcode | Call Number | Material Type | Item Category 1 | Status |
---|---|---|---|---|---|
Searching... | 30000010178134 | TP248.3 B684 2007 | Open Access Book | Book | Searching... |
Searching... | 30000003499211 | TP248.3 B684 2007 | Open Access Book | Book | Searching... |
On Order
Summary
Summary
If you are a process design, quality control, information systems, or auto-mation engineer in the biopharmaceutical, brewing, or bio-fuel industry, this handy resource will help you define, develop, and apply a virtual plant, model predictive control, first-principle models, neural networks, and multivariate statistical process control. The synergistic knowledge discovery on bench top or pilot plant scale can be ported to industrial scale processes. This learning process is consistent with the intent in the Process Analyzer and Process Control Tools sections of the FDA's ""Guidance for Industry PAT - A Framework for Innovative Pharmaceutical Development, Manufacturing and Quality Assurance.
Table of Contents
Acknowledgments | p. vii |
About the Authors | p. ix |
Preface | p. xi |
Chapter 1 Opportunities | p. 3 |
1-1 Introduction | p. 3 |
1-2 Analysis of Variability | p. 6 |
1-3 Transfer of Variability | p. 16 |
1-4 Online Indication of Performance | p. 24 |
1-5 Optimizing Performance | p. 27 |
1-6 Process Analytical Technology (PAT) | p. 28 |
References | p. 31 |
Chapter 2 Process Dynamics | p. 35 |
2-1 Introduction | p. 35 |
2-2 Performance Limits | p. 36 |
2-3 Self-Regulating Processes | p. 47 |
2-4 Integrating Processes | p. 51 |
References | p. 54 |
Chapter 3 Basic Feedback Control | p. 57 |
3-1 Introduction | p. 57 |
3-2 PID Modes, Structure, and Form | p. 60 |
3-3 PID Tuning | p. 71 |
3-4 Adaptive Control | p. 87 |
3-5 Set-Point Response Optimization | p. 91 |
References | p. 96 |
Chapter 4 Model Predictive Control | p. 99 |
4-1 Introduction | p. 99 |
4-2 Capabilities and Limitations | p. 100 |
4-3 Multiple Manipulated Variables | p. 109 |
4-4 Optimization | p. 116 |
References | p. 127 |
Chapter 5 Virtual Plant | p. 131 |
5-1 Introduction | p. 131 |
5-2 Key Features | p. 132 |
5-3 Spectrum of Uses | p. 138 |
5-4 Implementation | p. 141 |
References | p. 147 |
Chapter 6 First-Principle Models | p. 151 |
6-1 Introduction | p. 151 |
6-2 Our Location on the Model Landscape | p. 152 |
6-3 Mass, Energy, and Component Balances | p. 153 |
6-4 Heat of Reaction | p. 158 |
6-5 Charge Balance | p. 159 |
6-6 Parameters and Their Engineering Units | p. 162 |
6-7 Kinetics | p. 167 |
6-8 Mass Transfer | p. 180 |
6-9 Simulated Batch Profiles | p. 185 |
References | p. 188 |
Chapter 7 Neural Network Industrial Process Applications | p. 193 |
7-1 Introduction | p. 193 |
7-2 Types of Networks and Uses | p. 198 |
7-3 Training a Neural Network | p. 200 |
7-4 Timing Is Everything | p. 203 |
7-5 Network Generalization: More Isn't Always Better | p. 206 |
7-6 Network Development: Just How Do You Go about Developing a Network? | p. 208 |
7-7 Neural Network Example One | p. 211 |
7-8 Neural Network Example Two | p. 217 |
7-9 Designing Neural Network Control Systems | p. 233 |
7-10 Discussion and Future Direction | p. 235 |
7-11 Neural Network Point-Counterpoint | p. 239 |
References | p. 242 |
Chapter 8 Multivariate Statistical Process Control | p. 247 |
8-1 Introduction | p. 247 |
8-2 PCA Background | p. 249 |
8-3 Multiway PCA | p. 265 |
8-4 Model-based PCA (MB-PCA) | p. 272 |
8-5 Fault Detection | p. 276 |
References | p. 282 |
Appendix A Definition of Terms | p. 289 |
Appendix B Condition Number | p. 301 |
Appendix C Unification of Controller Tuning Relationships | p. 305 |
Appendix D Modern Myths | p. 317 |
Appendix E Enzyme Inactivity Decreased by Controlling the pH with a family of Bezier Curves [1] | p. 321 |
Index | p. 333 |