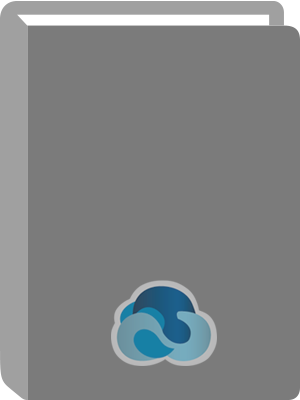
Available:*
Library | Item Barcode | Call Number | Material Type | Item Category 1 | Status |
---|---|---|---|---|---|
Searching... | 30000010126810 | QA76.87 I524 2001 | Open Access Book | Book | Searching... |
On Order
Summary
Summary
Independent Component Analysis (ICA) has recently become an important tool for modelling and understanding empirical datasets. It is a method of separating out independent sources from linearly mixed data, and belongs to the class of general linear models. ICA provides a better decomposition than other well-known models such as principal component analysis. This self-contained book contains a structured series of edited papers by leading researchers in the field, including an extensive introduction to ICA. The major theoretical bases are reviewed from a modern perspective, current developments are surveyed and many case studies of applications are described in detail. The latter include biomedical examples, signal and image denoising and mobile communications. ICA is discussed in the framework of general linear models, but also in comparison with other paradigms such as neural network and graphical modelling methods. The book is ideal for researchers and graduate students in the field.
Table of Contents
1 IntroductionStephen Roberts and Richard Everson |
2 Fast ICA by a fixed-point algorithm that maximizes non-GaussianityAapo Hyvarinen |
3 ICA, graphical models and variational methodsHagai Attias |
4 Nonlinear independent component analysisJuha Karhunen |
5 Separation of non-stationary natural signalsLucas Parra and Clay Spence |
6 Separation of non-stationary sources: algorithms and performanceJean-Francois Cardoso and Dinh-Tuan Pham |
7 Blind source separation by sparse decomposition in a signal dictionaryMichael Zibulevsky and Barak Pearlmutter and Pau Bofill and Pavel Kisilev |
8 Ensemble learning for blind source separationJames Miskin and David MacKay |
9 Image processing methods using ICA mixture modelsTe-Won Lee and Michael S. Lewicki |
10 Latent class and trait models for data classification and visualisationMark Girolami |
11 Particle filters for non-stationary ICARichard Everson and Stephen Roberts |
12 ICA: model order selection and dynamic source modelsWilliam Penny and Stephen Roberts and Richard Everson |