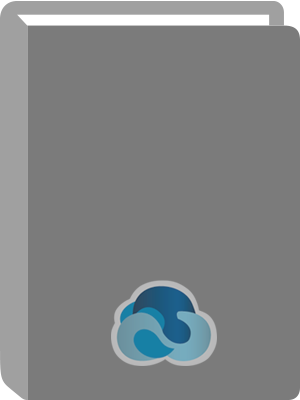
Available:*
Library | Item Barcode | Call Number | Material Type | Item Category 1 | Status |
---|---|---|---|---|---|
Searching... | 30000003685082 | TS156.8.L443 1996 | Open Access Book | Book | Searching... |
On Order
Summary
Summary
Explains the fundamentals of statistical process control (SPC) and how SPC tools work as feedback mechanisms and as a means of analyzing the causes of variation. Material on attributes and variables data, sampling, rational subgrouping, the components of variation, and measurement processes incorporates numerous case studies demonstrating how and where SPC concepts and techniques can be applied to real-life situations, and includes practice exercises and answers. For managers and engineers in industrial organizations. Annotation copyright by Book News, Inc., Portland, OR
Author Notes
Mary G. Leitnaker ( biography ) is a professor in the Statistics Department at the University of Tennessee.
Richard D. Sanders ( biography ) is a professor in the Statistics Department at the University of Tennessee.
Cheryl Hild ( biography ) is a research associate with the Management Development Center.
0201633906AB04062001
Excerpts
Excerpts
The objective of this book is to provide present and future managers and engineers of industrial organizations with the knowledge and information needed for the statistical study of the processes of an organization. To remain viable in an increasingly competitive world marketplace, organizations must be continually engaged in the study and improvement of the effectiveness and efficiency of their operations. It is the premise of this book that knowledge of variation is and will continue to be critical to the effective execution of these responsibilities. Crucial competencies required of managers and engineers in the future will be the ability to characterize the organization's processes with performance indicators that serve as guidance for improvement and to direct the organization in improvement of these measures. A conscious recognition of variation, an informed interpretation of the messages it contains, and an understanding of the effects variation has on process performance will help define the different behaviors and decisions that will characterize the future role of the manager and engineer. The topics in this book are intended to provide the statistical background necessary for the manager or engineer to assume these responsibilities. Presentation of the statistical methods and tools is supported by demonstration of correct use, practice, interpretation, and guidance for implementation. Clearly, a knowledge of statistics is insufficient by itself to provide the type of knowledge and guidance needed for process improvement. A critical component of improvement work is the management and technical knowledge brought to bear on determining where and how to employ the statistical techniques. The required knowledge of chemistry, physics, and of a host of applied engineering methods are not covered in this book. There is nothing directly on "people management skills." Instead, the book contains advice, based on observation and experience, as well as numerous case studies on the integration of statistical methods with management and engineering knowledge, which can lead to organizational improvement. Just as the roles of managers and engineers are changing in organizations, so are the uses of statistical methods in organizations. This change in the manner in which statistical methods are being used is reflected in recent writings by W. E. Deming (1986) and Ronald D. Moen, Thomas W. Nolan, and Lloyd P. Provost (1991) on enumerative and analytical methods in statistics. Enumerative methods, although primarily intended for studying a fixed population of items, have formed the bulk of statistical techniques taught at the undergraduate and graduate levels in statistics courses for managers and engineers. A perusal of the table of contents of most statistical textbooks designed for these audiences would verify this statement. Analytical statistical methods, on the other hand, are intended to provide insights into the causal mechanisms affecting the outcomes of ongoing processes. Processes occurring over time are subject to changes in environment, materials, methods, equipment, and other circumstances. It is the study of process behavior and how it is affected by these changing circumstances that is addressed by analytical studies. The demands for increased statistical skills for process investigation in the industrial world has had an effect on the content of statistical courses in universities and colleges and on professional education. There are a growing number of competent statistical books that present the basic formulas of statistical process control. In general, those books focus on the use of statistics as strict control or feedback mechanisms; they provide little demonstration of the use of these methods for the analytical studies required for identifying sources of variation. This book is intended not only to teach statistical techniques useful in such analytical studies, but also to illustrate how these techniques can be used in practice to understand the causal mechanisms that determine the output characteristics of processes and provide insights into the improvement of these processes. Some statistical theory is covered in an appendix. When used as a course text, the instructor can increase the value of the book by demonstrating the utility of theory in approaching a new situation, in searching for the correct technique, and by demonstrating and discussing the constraints often found in unspoken mathematical and process-based assumptions. Acknowledgments The examples and ideas in this textbook are primarily the result of the authors' association with the University of Tennessee's Management Development Center. Although too numerous to name, managers and engineers from industry who have attended U.T.'s executive education courses have provided numerous ideas, insights, and critiques of the thoughts in this book. The authors would like to express their appreciation to John Riblett, the center's director, for his considerable efforts in supporting the growth of faculty and ideas through his management of the center. Also, without the advice, support, and insights from our faculty colleagues at the center this book would not have been possible. 0201633906P04062001 Excerpted from The Power of Statistical Thinking: Improving Industrial Processes by Richard D. Sanders, Cheryl Hild, Mary G. Leitnaker All rights reserved by the original copyright owners. Excerpts are provided for display purposes only and may not be reproduced, reprinted or distributed without the written permission of the publisher.Table of Contents
1 Introduction to the Use of Statistical Methods in Strategic Organizational Improvement |
Process Definition |
Statistical Techniques for Process Study |
Managing Processes Within an Organizational Context |
The Impact of Variation on Achieving Process Objectives |
The Scientific Method and Process Management |
2 Tools for Process Study |
Use of Flowcharts for Describing Process Operations |
Planning for Data Collection |
Pareto Diagrams |
Cause and Effect Diagrams |
Introduction to the Concept of Statistical Control Charts |
Summary |
3 Control Charts for Attributes Data: p and np Charts |
Description of Attributes Data Requiring the Use of p or np Charts |
Construction of p Charts |
The Concept of Statistical Control |
Construction of np Charts |
Construction of p Charts When n Varies |
Collection of Attributes Data to Support Process Study: A Case Study |
Supporting Ideas for the Effective Use of p and np Charts |
Practice Problems |
Application Problem |
4 Control Charts for Attributes Data: c and u Charts |
Description of Attributes Data Requiring the Use of c or u Charts |
Construction of c Charts |
Construction of u Charts |
Using c and u Charts in Ongoing Process Improvement Work: A Case Study |
Supporting Ideas for the Effective Use of c and u Charts |
Summary |
Practice Problems |
Application Problem |
5 Control Charts for Variables Data: Variability and Location |
Description of Types of Variation in Process Data |
Identifying Variation and Knowing Its Sources |
Charts for Variables Data and Process Analysis |
Summaries of Process Behavior |
Examples of the Process Use of Range and X-bar Charts |
Supporting Principles for the Effective Use of R and X-bar Charts (or s and X-bar Charts) |
Practice Problems |
Application Problem |
6 Sampling and Subgrouping Principles |
Sampling, Subgrouping, and Elements of Purpose |
Chart Characteristics Direct Sampling and Subgroup Formation |
Three Sampling and Subgrouping Plans in a Dimensional Study |
Subgrouping Plans for Processes with Possible Fixed Differences |
Subgrouping Plans for Studying Coils, Webs, or Rolls |
Principles for Rational Subgrouping |
An Application of the Principles of Rational Subgrouping |
Summary |
Application Problems |
7 Control Charts for Variables Data: Moving Range and Individuals Charts |
Process Data with Logical Subgroups of Size One |
Evaluating Process Behavior with Moving Range and Individuals Charts |
A Discussion of Issues Associated with the Use of MR and X Charts |
Application of MR and X Charts in Process Study |
Ideas for the Effective Use of MR and X Charts |
Summary |
Practice Problems |
Application Problem |
8 Subgrouping and Components of Variation |
Understanding the Components Contributing to Total Variation |
Study of the Components of Variation in a Batch Process |
Example of a Multiple-level Subgrouping Plan and Analysis |
Study of Fixed Effects in a Hierarchical Model |
Practice Problems |
Application Problem |
9 Measurement Processes |
Characterizing a Measurement Process |
Evaluation of a Measurement Process: Reporting on Total Laboratory Variation |
The Effect of Measurement Variation on Process Study |
Comparison of Measurement Processes |
Gauge R and R Studies |
Recommendations for Ensuring Good Measurements |
Application Problems |
10 The Role of Designed Experiments in Process Management |
Definition of Designed Experiments |
Elements of Designed Experiments |
Basic Mechanics of Designed Experiments |
Additional Experimental Strategies |
Summary and Design References |
Practice Problems |
Appendix A Probability Models |
The Normal Probability Model |
The Binomial Probability Model: A Theoretical Basis for p and np Charts |
The Poisson Probability Model: A Theoretical Basis for c Charts |
Appendix B Runs Tests |
Seven Successive Points in or Beyond Zone C on Same Side of Centerline (or the Rule of Seven) |
Test for Length of Longest Run |
Test for Too Few Runs |
Two Out of Three Successive Points in Zone A or Beyond |
Four Out of Five Successive Points in Zone B or Beyond |
Fifteen or More Consecutive Points in Zone C on Either Side of Centerline |
Eight Consecutive Points Beyond Zone C on Either Side of Centerline |
Appendix C Symbols for More Detailed Process Flowcharts |
Appendix D Statistical Tables |
Table D.1 Standard Normal Distribution |
Table D.2 Factors for Use with X-bar and Range Charts |
Table D.3 Factors for Use with X-bar and s Charts |
Table D.4 Denominator Degrees of Freedom F(alpha) for alpha = .05 |
Table D.5 Denominator Degrees of Freedom F(alpha) for alpha = .01 |
Appendix E Answers to Practice Problems |
Bibliography |
Index |