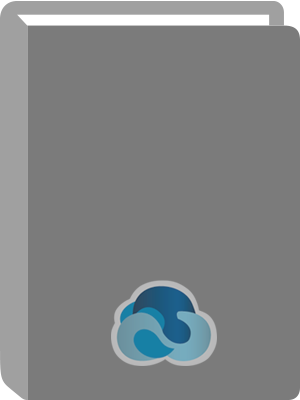
Available:*
Library | Item Barcode | Call Number | Material Type | Item Category 1 | Status |
---|---|---|---|---|---|
Searching... | 30000010267239 | CP 026145 | Computer File Accompanies Open Access Book | Compact Disc Accompanies Open Access Book | Searching... |
On Order
Summary
Summary
Autoregressive Conditional Heteroskedastic (ARCH) processes are used in finance to model asset price volatility over time. This book introduces both the theory and applications of ARCH models and provides the basic theoretical and empirical background, before proceeding to more advanced issues and applications. The Authors provide coverage of the recent developments in ARCH modelling which can be implemented using econometric software, model construction, fitting and forecasting and model evaluation and selection.
Key Features:
Presents a comprehensive overview of both the theory and the practical applications of ARCH, an increasingly popular financial modelling technique. Assumes no prior knowledge of ARCH models; the basics such as model construction are introduced, before proceeding to more complex applications such as value-at-risk, option pricing and model evaluation. Uses empirical examples to demonstrate how the recent developments in ARCH can be implemented. Provides step-by-step instructive examples, using econometric software, such as Econometric Views and the G@RCH module for the Ox software package, used in Estimating and Forecasting ARCH Models. Accompanied by a CD-ROM containing links to the software as well as the datasets used in the examples.Aimed at readers wishing to gain an aptitude in the applications of financial econometric modelling with a focus on practical implementation, via applications to real data and via examples worked with econometrics packages.
Author Notes
Evdokia Xekalaki, Department of Statistics, Athens University of Economics and Business
Professor Xekalaki has been teaching for nearly 30 years, and in that time has held such positions as Director of the graduate program, consultant to EUROSTAT and twice Chair of the Dept of Statistics at AUEB. She has published more than 50 papers in numerous international journals and has presented papers at many international conferences. She is also the Chief Editor of the journal Quality Technology and Quantitative Management and on the Editorial Board for the Journal of Applied Stochastic Models in Business and Industry.
Stavros Degiannakis, Department of Statistics, Athens University of Economics and Business
Adjunct lecturer in applied econometrics, Dr Degiannakis acquired his PhD last year, and has already had eight articles published in seven journals, and eight other papers presented at a variety of international conferences.
Table of Contents
Preface | p. xi |
Notation | p. xv |
1 What is an ARCH process? | p. 1 |
1.1 Introduction | p. 1 |
1.2 The autoregressive conditionally heteroscedastic process | p. 8 |
1.3 The leverage effect | p. 13 |
1.4 The non-trading period effect | p. 15 |
1.5 The non-synchronous trading effect | p. 15 |
1.6 The relationship between conditional variance and conditional mean | p. 16 |
1.6.1 The ARCH in mean model | p. 16 |
1.6.2 Volatility and serial correlation | p. 18 |
2 ARCH volatility specifications | p. 19 |
2.1 Model specifications | p. 19 |
2.2 Methods of estimation | p. 23 |
2.2.1 Maximum likelihood estimation | p. 23 |
2.2.2 Numerical estimation algorithms | p. 25 |
2.2.3 Quasi-maximum likelihood estimation | p. 28 |
2.2.4 Other estimation methods | p. 29 |
2.3 Estimating the GARCH model with EViews 6: an empirical example | p. 31 |
2.4 Asymmetric conditional volatility specifications | p. 42 |
2.5 Simulating ARCH models using EViews | p. 49 |
2.6 Estimating asymmetric ARCH models with G@RCH 4.2 OxMetrics: an empirical example | p. 55 |
2.7 Misspecification tests | p. 66 |
2.7.1 The Box-Pierce and Ljung-Box Q statistics | p. 66 |
2.7.2 Tse's residual based diagnostic test for conditional heteroscedasticity | p. 67 |
2.7.3 Engle's Lagrange multiplier test | p. 67 |
2.7.4 Engle and Ng's sign bias tests | p. 68 |
2.7.5 The Breusch-Pagan, Godfrey, Glejser, Harvey and White tests | p. 69 |
2.7.6 The Wald, likelihood ratio and Lagrange multiplier tests | p. 69 |
2.8 Other ARCH volatility specifications | p. 70 |
2.8.1 Regime-switching ARCH models | p. 70 |
2.8.2 Extended ARCH models | p. 72 |
2.9 Other methods of volatility modelling | p. 76 |
2.10 Interpretation of the ARCH process | p. 82 |
Appendix | p. 86 |
3 Fractionally integrated ARCH models | p. 107 |
3.1 Fractionally integrated ARCH model specifications | p. 107 |
3.2 Estimating fractionally integrated ARCH models using G@RCH 4.2 OxMetrics: an empirical example | p. 111 |
3.3 A more detailed investigation of the normality of the standardized residuals: goodness-of-fit tests | p. 122 |
3.3.1 EDF tests | p. 123 |
3.3.2 Chi-square tests | p. 124 |
3.3.3 QQ plots | p. 125 |
3.3.4 Goodness-of-fit tests using EViews and G@RCH | p. 126 |
Appendix | p. 129 |
4 Volatility forecasting: an empirical example using EViews 6 | p. 143 |
4.1 One-step-ahead volatility forecasting | p. 143 |
4.2 Ten-step-ahead volatility forecasting | p. 150 |
Appendix | p. 154 |
5 Other distributional assumptions | p. 163 |
5.1 Non-normally distributed standardized innovations | p. 163 |
5.2 Estimating ARCH models with non-normally distributed standardized innovations using G@RCH 4.2 OxMetrics: an empirical example | p. 168 |
5.3 Estimating ARCH models with non-normally distributed standardized innovations using EViews 6: an empirical example | p. 174 |
5.4 Estimating ARCH models with non-normally distributed standardized innovations using EViews 6: the logl object | p. 176 |
Appendix | p. 182 |
6 Volatility forecasting: an empirical example using G@RCH Ox | p. 185 |
Appendix | p. 195 |
7 Intraday realized volatility models | p. 217 |
7.1 Realized volatility | p. 217 |
7.2 Intraday volatility models | p. 220 |
7.3 Intraday realized volatility and ARFIMAX models in G@RCH 4.2 OxMetrics: an empirical example | p. 223 |
7.3.1 Descriptive statistics | p. 223 |
7.3.2 In-sample analysis | p. 228 |
7.3.3 Out-of-sample analysis | p. 232 |
8 Applications in value-at-risk, expected shortfall and options pricing | p. 239 |
8.1 One-day-ahead value-at-risk forecasting | p. 239 |
8.1.1 Value-at-risk | p. 239 |
8.1.2 Parametric value-at-risk modelling | p. 240 |
8.1.3 Intraday data and value-at-risk modelling | p. 242 |
8.1.4 Non-parametric and semi-parametric value-at-risk modelling | p. 244 |
8.1.5 Back-testing value-at-risk | p. 245 |
8.1.6 Value-at-risk loss functions | p. 248 |
8.2 One-day-ahead expected shortfall forecasting | p. 248 |
8.2.1 Historical simulation and filtered historical simulation for expected shortfall | p. 251 |
8.2.2 Loss functions for expected shortfall | p. 251 |
8.3 FTSE100 index: one-step-ahead value-at-risk and expected shortfall forecasting | p. 252 |
8.4 Multi-period value-at-risk and expected shortfall forecasting | p. 258 |
8.5 ARCH volatility forecasts in Black-Scholes option pricing | p. 260 |
8.5.1 Options | p. 261 |
8.5.2 Assessing the performance of volatility forecasting methods | p. 269 |
8.5.3 Black-Scholes option pricing using a set of ARCH processes | p. 270 |
8.5.4 Trading straddles based on a set of ARCH processes | p. 271 |
8.5.5 Discussion | p. 279 |
8.6 ARCH option pricing formulas | p. 281 |
8.6.1 Computaion of Duan's ARCH option prices: an example | p. 286 |
Appendix | p. 288 |
9 Implied volatility indices and ARCH models | p. 341 |
9.1 Implied volatility | p. 341 |
9.2 The VIX index | p. 342 |
9.3 The implied volatility index as an explanatory variable | p. 344 |
9.4 ARFIMAX model for implied volatility index | p. 349 |
Appendix | p. 352 |
10 ARCH model evaluation and selection | p. 357 |
10.1 Evaluation of ARCH models | p. 358 |
10.1.1 Model evaluation viewed in terms of information criteria | p. 359 |
10.1.2 Model evaluation viewed in terms of statistical loss functions | p. 360 |
10.1.3 Consistent ranking | p. 367 |
10.1.4 Simulation, estimation and evaluation | p. 377 |
10.1.5 Point, interval and density forecasts | p. 383 |
10.1.6 Model evaluation viewed in terms of loss functions based on the use of volatility forecasts | p. 384 |
10.2 Selection of ARCH models | p. 386 |
10.2.1 The Diebold-Mariano test | p. 386 |
10.2.2 The Harvey-Leybourne-Newbold test | p. 389 |
10.2.3 The Morgan-Granger-Newbold test | p. 389 |
10.2.4 White's reality check for data snooping | p. 390 |
10.2.5 Hansen's superior predictive ability test | p. 390 |
10.2.6 The standardized prediction error criterion | p. 393 |
10.2.7 Forecast encompassing tests | p. 400 |
10.3 Application of loss functions as methods of model selection | p. 401 |
10.3.1 Applying the SPEC model selection method | p. 401 |
10.3.2 Applying loss functions as methods of model selection | p. 402 |
10.3.3 Median values of loss functions as methods of model selection | p. 407 |
10.4 The SPA test for VaR and expected shortfall | p. 408 |
Appendix | p. 410 |
11 Multivariate ARCH models | p. 445 |
11.1 Model Specifications | p. 446 |
11.1.1 Symmetric model specifications | p. 446 |
11.1.2 Asymmetric and long-memory model specifications | p. 453 |
11.2 Maximum likelihood estimation | p. 454 |
11.3 Estimating multivariate ARCH models using EViews 6 | p. 456 |
11.4 Estimating multivariate ARCH models using G@RCH 5.0 | p. 465 |
11.5 Evaluation of multivariate ARCH models | p. 473 |
Appendix | p. 475 |
References | p. 479 |
Author Index | p. 521 |
Subject Index | p. 533 |