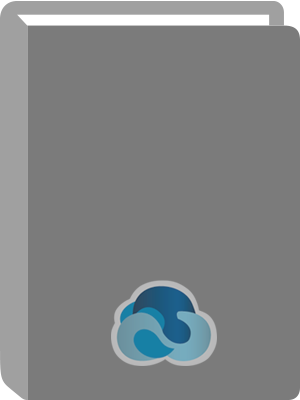
Available:*
Library | Item Barcode | Call Number | Material Type | Item Category 1 | Status |
---|---|---|---|---|---|
Searching... | 30000010119031 | QA76.9.D3 B374 2006 | Open Access Book | Book | Searching... |
Searching... | 30000010155763 | QA76.9.D3 B374 2006 | Open Access Book | Book | Searching... |
On Order
Summary
Summary
Poor data quality can seriously hinder or damage the efficiency and effectiveness of organizations and businesses. The growing awareness of such repercussions has led to major public initiatives like the "Data Quality Act" in the USA and the "European 2003/98" directive of the European Parliament.
Batini and Scannapieco present a comprehensive and systematic introduction to the wide set of issues related to data quality. They start with a detailed description of different data quality dimensions, like accuracy, completeness, and consistency, and their importance in different types of data, like federated data, web data, or time-dependent data, and in different data categories classified according to frequency of change, like stable, long-term, and frequently changing data. The book's extensive description of techniques and methodologies from core data quality research as well as from related fields like data mining, probability theory, statistical data analysis, and machine learning gives an excellent overview of the current state of the art. The presentation is completed by a short description and critical comparison of tools and practical methodologies, which will help readers to resolve their own quality problems.
This book is an ideal combination of the soundness of theoretical foundations and the applicability of practical approaches. It is ideally suited for everyone - researchers, students, or professionals - interested in a comprehensive overview of data quality issues. In addition, it will serve as the basis for an introductory course or for self-study on this topic.
Author Notes
Carlo Batini is full professor of Computer Engineering at University of Milano Bicocca. He has been associate professor since 1983 and full professor since 1986. His research interests include cooperative information systems, information systems and data base modeling and design, usability of information systems, data and information quality. From 1995 to 2003 he was a member of the board of directors of the Authority for Information Technology in public administration, where he headed several large scale projects for the modernization of public administration.
Monica Scannapieco is a research associate at the Computer Engineering Department of the University of Roma La Sapienza. Her research interests are data quality issues, including data quality dimensions, measurement and improvement techniques, dynamics of data quality, record matching.
Table of Contents
1 Introduction to Data Quality | p. 1 |
1.1 Why Data Quality is Relevant | p. 1 |
1.2 Introduction to the Concept of Data Quality | p. 4 |
1.3 Data Quality and Types of Data | p. 6 |
1.4 Data Quality and Types of Information Systems | p. 9 |
1.5 Main Research Issues and Application Domains in Data Quality | p. 11 |
1.5.1 Research Issues in Data Quality | p. 12 |
1.5.2 Application Domains in Data Quality | p. 12 |
1.5.3 Research Areas Related to Data Quality | p. 16 |
1.6 Summary | p. 17 |
2 Data Quality Dimensions | p. 19 |
2.1 Accuracy | p. 20 |
2.2 Completeness | p. 23 |
2.2.1 Completeness of Relational Data | p. 24 |
2.2.2 Completeness of Web Data | p. 27 |
2.3 Time-Related Dimensions: Currency, Timeliness, and Volatility | p. 28 |
2.4 Consistency | p. 30 |
2.4.1 Integrity Constraints | p. 30 |
2.4.2 Data Edits | p. 31 |
2.5 Other Data Quality Dimensions | p. 32 |
2.5.1 Accessibility | p. 34 |
2.5.2 Quality of Information Sources | p. 35 |
2.6 Approaches to the Definition of Data Quality Dimensions | p. 36 |
2.6.1 Theoretical Approach | p. 36 |
2.6.2 Empirical Approach | p. 38 |
2.6.3 Intuitive Approach | p. 39 |
2.6.4 A Comparative Analysis of the Dimension Definitions | p. 39 |
2.6.5 Trade-offs Between Dimensions | p. 40 |
2.7 Schema Quality Dimensions | p. 42 |
2.7.1 Readability | p. 45 |
2.7.2 Normalization | p. 45 |
2.8 Summary | p. 48 |
3 Models for Data Quality | p. 51 |
3.1 Introduction | p. 51 |
3.2 Extensions of Structured Data Models | p. 52 |
3.2.1 Conceptual Models | p. 52 |
3.2.2 Logical Models for Data Description | p. 54 |
3.2.3 The Polygen Model for Data Manipulation | p. 55 |
3.2.4 Data Provenance | p. 56 |
3.3 Extensions of Semistructured Data Models | p. 59 |
3.4 Management Information System Models | p. 61 |
3.4.1 Models for Process Description: the IP-MAP model | p. 61 |
3.4.2 Extensions of IP-MAP | p. 62 |
3.4.3 Data Models | p. 64 |
3.5 Summary | p. 68 |
4 Activities and Techniques for Data Quality: Generalities | p. 69 |
4.1 Data Quality Activities | p. 70 |
4.2 Quality Composition | p. 71 |
4.2.1 Models and Assumptions | p. 74 |
4.2.2 Dimensions | p. 76 |
4.2.3 Accuracy | p. 78 |
4.2.4 Completeness | p. 79 |
4.3 Error Localization and Correction | p. 82 |
4.3.1 Localize and Correct Inconsistencies | p. 82 |
4.3.2 Incomplete Data | p. 85 |
4.3.3 Discovering Outliers | p. 86 |
4.4 Cost and Benefit Classifications | p. 88 |
4.4.1 Cost Classifications | p. 89 |
4.4.2 Benefits Classification | p. 94 |
4.5 Summary | p. 95 |
5 Object Identification | p. 97 |
5.1 Historical Perspective | p. 98 |
5.2 Object Identification for Different Data Types | p. 99 |
5.3 The High-Level Process for Object Identification | p. 101 |
5.4 Details on the Steps for Object Identification | p. 103 |
5.4.1 Preprocessing | p. 103 |
5.4.2 Search Space Reduction | p. 104 |
5.4.3 Comparison Functions | p. 104 |
5.5 Object Identification Techniques | p. 106 |
5.6 Probabilistic Techniques | p. 106 |
5.6.1 The Fellegi and Sunter Theory and Extensions | p. 107 |
5.6.2 A Cost-Based Probabilistic Technique | p. 112 |
5.7 Empirical Techniques | p. 113 |
5.7.1 Sorted Neighborhood Method and Extensions | p. 113 |
5.7.2 The Priority Queue Algorithm | p. 116 |
5.7.3 A Technique for Complex Structured Data: Delphi | p. 117 |
5.7.4 XML Duplicate Detection: DogmatiX | p. 119 |
5.7.5 Other Empirical Methods | p. 120 |
5.8 Knowledge-Based Techniques | p. 121 |
5.8.1 A Rule-Based Approach: Intelliclean | p. 122 |
5.8.2 Learning Methods for Decision Rules: Atlas | p. 123 |
5.9 Comparison of Techniques | p. 125 |
5.9.1 Metrics | p. 125 |
5.9.2 Search Space Reduction Methods | p. 127 |
5.9.3 Comparison Functions | p. 127 |
5.9.4 Decision Methods | p. 128 |
5.9.5 Results | p. 130 |
5.10 Summary | p. 131 |
6 Data Quality Issues in Data Integration Systems | p. 133 |
6.1 Introduction | p. 133 |
6.2 Generalities on Data Integration Systems | p. 134 |
6.2.1 Query Processing | p. 135 |
6.3 Techniques for Quality-Driven Query Processing | p. 137 |
6.3.1 The QP-alg: Quality-Driven Query Planning | p. 138 |
6.3.2 DaQuinCIS Query Processing | p. 140 |
6.3.3 Fusionplex Query Processing | p. 141 |
6.3.4 Comparison of Quality-Driven Query Processing Techniques | p. 143 |
6.4 Instance-level Conflict Resolution | p. 143 |
6.4.1 Classification of Instance-Level Conflicts | p. 144 |
6.4.2 Overview of Techniques | p. 146 |
6.4.3 Comparison of Instance-level Conflict Resolution Techniques | p. 156 |
6.5 Inconsistencies in Data Integration: a Theoretical Perspective | p. 157 |
6.5.1 A Formal Framework for Data Integration | p. 157 |
6.5.2 The Problem of Inconsistency | p. 158 |
6.6 Summary | p. 160 |
7 Methodologies for Data Quality Measurement and Improvement | p. 161 |
7.1 Basics on Data Quality Methodologies | p. 161 |
7.1.1 Inputs and Outputs | p. 161 |
7.1.2 Classification of Methodologies | p. 164 |
7.1.3 Comparison among Data-driven and Process-driven Strategies | p. 164 |
7.2 Assessment Methodologies | p. 167 |
7.3 Comparative Analysis of General-purpose Methodologies | p. 170 |
7.3.1 Basic Common Phases Among Methodologies | p. 171 |
7.3.2 The TDQM Methodology | p. 172 |
7.3.3 The TQdM Methodology | p. 174 |
7.3.4 The Istat Methodology | p. 177 |
7.3.5 Comparisons of Methodologies | p. 180 |
7.4 The CDQM methodology | p. 181 |
7.4.1 Reconstruct the State of Data | p. 182 |
7.4.2 Reconstruct Business Processes | p. 183 |
7.4.3 Reconstruct Macroprocesses and Rules | p. 183 |
7.4.4 Check Problems with Users | p. 184 |
7.4.5 Measure Data Quality | p. 184 |
7.4.6 Set New Target DQ Levels | p. 185 |
7.4.7 Choose Improvement Activities | p. 186 |
7.4.8 Choose Techniques for Data Activities | p. 187 |
7.4.9 Find Improvement Processes | p. 187 |
7.4.10 Choose the Optimal Improvement Process | p. 188 |
7.5 A Case Study in the e-Government Area | p. 188 |
7.6 Summary | p. 199 |
8 Tools for Data Quality | p. 201 |
8.1 Introduction | p. 201 |
8.2 Tools | p. 202 |
8.2.1 Potter's Wheel | p. 203 |
8.2.2 Telcordia's Tool | p. 205 |
8.2.3 Ajax | p. 206 |
8.2.4 Artkos | p. 208 |
8.2.5 Choice Maker | p. 210 |
8.3 Frameworks for Cooperative Information Systems | p. 212 |
8.3.1 DaQuinCIS Framework | p. 212 |
8.3.2 FusionPlex Framework | p. 215 |
8.4 Toolboxes to Compare Tools | p. 216 |
8.4.1 Theoretical Approach | p. 216 |
8.4.2 Tailor | p. 217 |
8.5 Summary | p. 218 |
9 Open Problems | p. 221 |
9.1 Dimensions and Metrics | p. 221 |
9.2 Object Identification | p. 222 |
9.2.1 XML Object Identification | p. 223 |
9.2.2 Object Identification of Personal Information | p. 224 |
9.2.3 Record Linkage and Privacy | p. 225 |
9.3 Data Integration | p. 227 |
9.3.1 Trust-Aware Query Processing in P2P Contexts | p. 227 |
9.3.2 Cost-Driven Query Processing | p. 228 |
9.4 Methodologies | p. 230 |
9.5 Conclusions | p. 235 |
References | p. 237 |
Index | p. 249 |