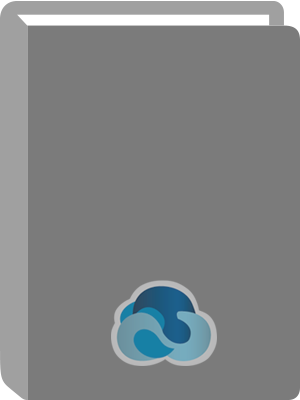
Available:*
Library | Item Barcode | Call Number | Material Type | Item Category 1 | Status |
---|---|---|---|---|---|
Searching... | 30000010296486 | QA279.5 K78 2011 | Open Access Book | Book | Searching... |
On Order
Summary
Summary
There is an explosion of interest in Bayesian statistics, primarily because recently created computational methods have finally made Bayesian analysis tractable and accessible to a wide audience. Doing Bayesian Data Analysis, A Tutorial Introduction with R and BUGS, is for first year graduate students or advanced undergraduates and provides an accessible approach, as all mathematics is explained intuitively and with concrete examples. It assumes only algebra and 'rusty' calculus. Unlike other textbooks, this book begins with the basics, including essential concepts of probability and random sampling. The book gradually climbs all the way to advanced hierarchical modeling methods for realistic data. The text provides complete examples with the R programming language and BUGS software (both freeware), and begins with basic programming examples, working up gradually to complete programs for complex analyses and presentation graphics. These templates can be easily adapted for a large variety of students and their own research needs.The textbook bridges the students from their undergraduate training into modern Bayesian methods.
Author Notes
John K. Kruschke is Professor of Psychological and Brain Sciences, and Adjunct Professor of Statistics, at Indiana University in Bloomington, Indiana, USA. He is eight-time winner of Teaching Excellence Recognition Awards from Indiana University. He won the Troland Research Award from the National Academy of Sciences (USA), and the Remak Distinguished Scholar Award from Indiana University. He has been on the editorial boards of various scientific journals, including Psychological Review, the Journal of Experimental Psychology: General, and the Journal of Mathematical Psychology, among others.
After attending the Summer Science Program as a high school student and considering a career in astronomy, Kruschke earned a bachelor's degree in mathematics (with high distinction in general scholarship) from the University of California at Berkeley. As an undergraduate, Kruschke taught self-designed tutoring sessions for many math courses at the Student Learning Center. During graduate school he attended the 1988 Connectionist Models Summer School, and earned a doctorate in psychology also from U.C. Berkeley. He joined the faculty of Indiana University in 1989. Professor Kruschke's publications can be found at his Google Scholar page. His current research interests focus on moral psychology.
Professor Kruschke taught traditional statistical methods for many years until reaching a point, circa 2003, when he could no longer teach corrections for multiple comparisons with a clear conscience. The perils of p values provoked him to find a better way, and after only several thousand hours of relentless effort, the 1st and 2nd editions of Doing Bayesian Data Analysis emerged.
Table of Contents
This Book's Organization: Read me First! |
The Basics: Parameters, Probability, Bayes' Rule and R |
What is this stuff called probability? |
Bayes' Rule |
Part II All the Fundamental Concepts and Techniques in a Simple Scenario |
Inferring a Binomial Proportion via Exact mathematical Analysis |
Inferring a Binomial Proportion via Grid Approximation |
Inferring a Binomial Proportion via Monte Carlo Methods |
Inferences Regarding Two Binomial Proportions |
Bernoulli Likelihood with Hierarchical Prior |
Hierarchical modeling and model comparison |
Null Hypothesis Significance Testing |
Bayesian Approaches to Testing a Point ("Null") Hypothesis |
Goals, Power, and Sample Size |
Part III The Generalized Linear Model |
Overview of the Generalized Linear Model |
Metric Predicted Variable on a Single Group |
Metric Predicted Variable with One Metric Predictor |
Metric Predicted Variable with Multiple Metric Predictors |
Metric Predicted Variable with One Nominal Predictor |
Metric Predicted Variable with Multiple Nominal Predictors |
Dichotomous Predicted Variable |
Original Predicted Variable, Contingency Table Analysis |
Part IV Tools in the Trunk |
Reparameterization, a.k.a. Change of Variables |
References |
Index |