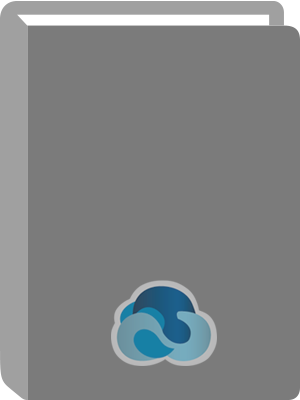
Available:*
Library | Item Barcode | Call Number | Material Type | Item Category 1 | Status |
---|---|---|---|---|---|
Searching... | 30000010160377 | QA401 M62 2007 | Open Access Book | Book | Searching... |
On Order
Summary
Summary
This Festschrift is intended as a homage to our esteemed colleague, friend and maestro Giorgio Picci on the occasion of his sixty-?fth birthday. We have knownGiorgiosince our undergraduatestudies at the University of Padova, wherewe ?rst experiencedhisfascinatingteachingin theclass ofSystem Identi?cation. While progressing through the PhD program, then continuing to collaborate with him and eventually becoming colleagues, we have had many opportunitiesto appreciate the value of Giorgio as a professor and a scientist, and chie?y as a person. We learned a lot from him and we feel indebted for his scienti?c guidance, his constant support, encouragement and enthusiasm. For these reasons we are proud to dedicate this book to Giorgio. The articles in the volume will be presented by prominent researchers at the "- ternational Conference on Modeling, Estimation and Control: A Symposium in Honor of Giorgio Picci on the Occasion of his Sixty-Fifth Birthday", to be held in Venice on October 4-5, 2007. The material covers a broad range of topics in mathematical systems theory, esti- tion, identi?cation and control, re?ecting the wide network of scienti?c relationships established during the last thirty years between the authors and Giorgio. Critical d- cussion of fundamental concepts, close collaboration on speci?c topics, joint research programs in this group of talented people have nourished the development of the ?eld, where Giorgio has contributed to establishing several cornerstones.
Table of Contents
Coefficients of Variations in Analysis of Macro-policy Effects: An Example of Two-Parameter Poisson-Dirichlet Distributions | p. 1 |
1 Introduction | p. 1 |
2 The Model | p. 2 |
3 Asymptotic Properties of the Number of Sectors | p. 2 |
4 The Coefficients of Variation | p. 3 |
4.1 The Number of Sectors | p. 3 |
4.2 The Number of Sectors of Specified Size | p. 3 |
5 Discussion | p. 3 |
References | p. 4 |
How Many Experiments Are Needed to Adapt? | p. 5 |
1 Introduction | p. 5 |
2 Worst-Case Approach to Adaptation | p. 7 |
2.1 Worst-Case Performance | p. 7 |
2.2 Adaptive Design | p. 8 |
3 The Experimental Effort Needed for Adaptation | p. 9 |
4 A Numerical Example | p. 11 |
5 Conclusions | p. 13 |
References | p. 14 |
A Mutual Information Based Distance for Multivariate Gaussian Processes | p. 15 |
1 Introduction | p. 15 |
2 Model Class | p. 17 |
3 Principal Angles, Canonical Correlations and Mutual Information | p. 19 |
3.1 Principal Angles and Directions | p. 20 |
3.2 Canonical Correlations | p. 20 |
3.3 Mutual Information | p. 21 |
3.4 Application to Stochastic Processes | p. 21 |
4 A Distance Between Multivariate Gaussian Processes | p. 25 |
4.1 Definition and Metric Properties | p. 25 |
4.2 Computation | p. 26 |
5 Special Case of Scalar Processes | p. 26 |
5.1 Relation with Subspace Angles Between Scalar Stochastic Processes | p. 27 |
5.2 Relation with a Cepstral Distance | p. 27 |
6 The Cepstral Nature of the Mutual Information Distance | p. 28 |
6.1 Multivariate Power Cepstrum and Cepstral Distance | p. 28 |
6.2 The Cepstral Nature of the Mutual Information Distance | p. 29 |
7 Conclusions and Open Problems | p. 30 |
7.1 Conclusions | p. 30 |
7.2 Open Problems | p. 31 |
References | p. 31 |
Differential Forms and Dynamical Systems | p. 35 |
1 Introduction | p. 35 |
2 Planar Dynamical Systems | p. 36 |
3 The Principle of the Torus for Autonomous Systems | p. 40 |
4 Lyapunov-Like Differential Forms for the Existence of Cross Sections | p. 41 |
5 Necessary and Sufficient Conditions for Existence of Periodic Orbits | p. 42 |
6 Stability and Robustness of Periodic Orbits | p. 43 |
References | p. 44 |
An Algebraic Framework for Bayes Nets of Time Series | p. 45 |
1 Introduction | p. 45 |
2 Conditional Independence and Stochastic Realization | p. 46 |
3 Lattice Conditionally Independence and Stochastic Realization | p. 48 |
3.1 Lattices of Subspaces | p. 48 |
3.2 Lattice Conditionally Orthogonal Stochastic Hilbert Spaces | p. 49 |
4 Spatially Patterned Infinite Bayes Nets | p. 53 |
References | p. 56 |
A Birds Eye View on System Identification | p. 59 |
1 Introduction | p. 59 |
2 Structure Theory | p. 61 |
3 Estimation for a Given Subclass | p. 64 |
4 Model Selection | p. 67 |
5 Linear Non-mainstream Cases | p. 68 |
6 Nonlinear Systems | p. 69 |
7 Present State and Future Developments | p. 69 |
References | p. 70 |
Further Results on the Byrnes-Georgiou-Lindquist Generalized Moment Problem | p. 73 |
1 Introduction | p. 73 |
2 A Generalized Moment Problem | p. 74 |
3 Kullback-Leibler Criterion | p. 75 |
4 Optimality Conditions and the Dual Problem | p. 76 |
5 An Existence Theorem | p. 77 |
6 A Descent Method for the Dual Problem | p. 79 |
References | p. 81 |
Factor Analysis and Alternating Minimization | p. 85 |
1 Introduction | p. 85 |
2 The Model | p. 86 |
3 Lifting of the Original Problem | p. 87 |
3.1 The First Partial Minimization Problem | p. 88 |
3.2 The Second Partial Minimization Problem | p. 89 |
3.3 The Link to the Original Problem | p. 91 |
4 Alternating Minimization Algorithm | p. 92 |
4.1 The Algorithm | p. 92 |
4.2 Proof of Proposition 1 | p. 94 |
References | p. 95 |
A Appendix | p. 95 |
A.1 Multivariate Normal Distribution | p. 95 |
A.2 Partitioned Matrices | p. 95 |
Tensored Polynomial Models | p. 97 |
1 Introduction | p. 97 |
2 Tensored Models | p. 98 |
2.1 Preliminaries | p. 98 |
2.2 Tensored Polynomial and Rational Models | p. 99 |
2.3 Module Structures on Tensored Models | p. 101 |
2.4 Duality | p. 103 |
2.5 Homomorphisms of Tensored Models | p. 104 |
3 Applications | p. 105 |
3.1 The Space of Intertwining Maps | p. 105 |
3.2 The Polynomial Sylvester Equation | p. 106 |
3.3 Solving the Sylvester Equation | p. 107 |
3.4 Invariant Factors of the Sylvester Map | p. 109 |
References | p. 112 |
Distances Between Time-Series and Their Autocorrelation Statistics | p. 113 |
1 Introduction | p. 113 |
2 Interpretation of the L[subscript 1] Distance | p. 114 |
3 A Distance Between Covariance Matrices | p. 115 |
3.1 An Example | p. 118 |
4 Approximating Sample Covariances | p. 120 |
4.1 Comparison with the von Neumann Entropy | p. 120 |
4.2 Structured Covariances | p. 121 |
References | p. 122 |
Global Identifiability of Complex Models, Constructed from Simple Submodels | p. 123 |
1 Introduction | p. 123 |
2 The Problem | p. 124 |
3 A Simple Example of Interconnected Modules | p. 126 |
4 Preliminary Considerations and Tools | p. 127 |
5 Identifiability Analysis | p. 128 |
6 A Formal Theorem on Identifiability from Sub-models | p. 131 |
6.1 Global Identifiability | p. 131 |
6.2 Local Identifiability | p. 132 |
7 Conclusions | p. 132 |
References | p. 133 |
Identification of Hidden Markov Models - Uniform LLN-s | p. 135 |
1 Introduction | p. 135 |
2 Hidden Markov Models | p. 136 |
3 L-Mixing Processes | p. 138 |
4 Asymptotic Properties of the Log-Likelihood Function | p. 139 |
5 The Case of Primitive Q-s | p. 141 |
6 The Derivative of the Predictive Filter | p. 144 |
7 Uniform Laws of Large Numbers | p. 147 |
8 Estimation of Hidden Markov Models | p. 149 |
References | p. 149 |
Identifiability and Informative Experiments in Open and Closed-Loop Identification | p. 151 |
1 Introduction | p. 151 |
2 The Prediction Error Identification Setup | p. 153 |
3 Identifiability, Informative Data, and All That Jazz | p. 154 |
4 Analysis of the Information Matrix | p. 157 |
4.1 Expressions of the Pseudoregression Vector | p. 157 |
4.2 The Range and Kernel of Rank-One Vector Processes | p. 158 |
4.3 Regularity Conditions for I([theta]): A First Analysis | p. 159 |
4.4 Rich and Exciting Signals | p. 161 |
5 Regularity of I([theta]) for ARMAX and BJ Model Structures | p. 166 |
6 Conclusions | p. 169 |
References | p. 169 |
On Interpolation and the Kimura-Georgiou Parametrization | p. 171 |
1 Introduction | p. 171 |
2 Interpolation Conditions as Matrix Equations | p. 172 |
3 The Connection with the Kimura-Georgiou Parametrization | p. 177 |
References | p. 182 |
The Control of Error in Numerical Methods | p. 183 |
1 Introduction | p. 183 |
2 A Simple Example | p. 184 |
3 Four-Step Adams-Bashforth | p. 186 |
4 Statistical Analysis | p. 190 |
5 Conclusion | p. 192 |
References | p. 192 |
Contour Reconstruction and Matching Using Recursive Smoothing Splines | p. 193 |
1 Introduction | p. 193 |
2 Problem Formulation and Motivation | p. 194 |
3 Some Theoretical Properties | p. 196 |
3.1 Proper Periodicity Conditions | p. 196 |
3.2 Continuous Time, Continuous Data | p. 197 |
3.3 Continuous Time, Discrete Data | p. 199 |
3.4 Continuous Time, Discrete Data Iterated | p. 200 |
4 Data Set Reconstruction | p. 200 |
5 Evaluation of Recursive Spline Method | p. 202 |
6 Conclusions | p. 205 |
References | p. 206 |
Role of LQ Decomposition in Subspace Identification Methods | p. 207 |
1 Introduction | p. 207 |
2 State-Input-Output Matrix Equation | p. 208 |
3 MOESP Method | p. 209 |
4 N4SID Method | p. 211 |
4.1 Zero-Input Responses | p. 211 |
4.2 Relation to Ho-Kalman's Method | p. 214 |
4.3 State Vector and Zero-State Response | p. 214 |
4.4 Zero-State Response | p. 215 |
5 Conclusions | p. 216 |
References | p. 217 |
Canonical Operators on Graphs | p. 221 |
1 Introduction | p. 221 |
2 Graphs | p. 222 |
2.1 The Geometry of Graphs and Digraphs | p. 222 |
2.2 Operator Theory on Graphs and Digraphs | p. 224 |
3 Differences, Divergences, Laplacians and Dirac Operators | p. 227 |
4 Operators on Weighted Graphs | p. 230 |
5 The Incidence Operator and Its Kin | p. 232 |
6 The Drift of a Digraph | p. 235 |
References | p. 236 |
Prediction-Error Approximation by Convex Optimization | p. 239 |
1 Introduction | p. 239 |
2 Prediction-Error Approximation | p. 240 |
3 Prediction-Error Approximation in Restricted Model Classes | p. 241 |
4 The Kullback-Leibler Criterion and Maximum-Likelihood Identification | p. 245 |
5 Prediction-Error Approximation by Analytic Interpolation | p. 246 |
6 Conclusion | p. 248 |
References | p. 248 |
Patchy Solutions of Hamilton-Jacobi-Bellman Partial Differential Equations | p. 251 |
1 Hamilton Jacobi Bellman PDEs | p. 251 |
2 Other Approaches | p. 255 |
3 New Approach | p. 255 |
4 One Dimensional HJB PDEs | p. 256 |
5 One Dimensional Example | p. 260 |
6 HJB PDEs in Higher Dimensions | p. 261 |
7 Two Dimensional Example | p. 268 |
8 Conclusion | p. 269 |
References | p. 269 |
A Geometric Assignment Problem for Robotic Networks | p. 271 |
1 Introduction | p. 271 |
2 Geometric and Stochastic Preliminaries | p. 272 |
2.1 The Euclidean Traveling Salesperson Problem | p. 273 |
2.2 Bins and Balls | p. 273 |
2.3 Random Geometric Graphs | p. 273 |
3 Network Model and Problem Statement | p. 274 |
3.1 Robotic Network Model | p. 274 |
3.2 The Target Assignment Problem | p. 274 |
3.3 Sparse and Dense Environments | p. 275 |
4 Sparse Environments | p. 275 |
4.1 Assignment-Based Algorithms with Lower Bound Analysis | p. 275 |
4.2 The ETSP AssgmtAlgorithm with Upper Bound Analysis | p. 276 |
5 Dense Environments | p. 279 |
5.1 The Grid AssgmtAlgorithm with Complexity Analysis | p. 279 |
5.2 A Sensor Based Version | p. 282 |
5.3 Congestion Issues | p. 282 |
6 Conclusion and Extensions | p. 283 |
References | p. 283 |
On the Distance Between Non-stationary Time Series | p. 285 |
1 Introduction | p. 285 |
2 Formalization | p. 286 |
2.1 Introducing Nuisances | p. 287 |
2.2 Dynamic Time Warping | p. 288 |
2.3 Dynamics, or Lack Thereof, in DTW | p. 289 |
3 Time Warping Under Dynamic Constraints | p. 290 |
3.1 Going Blind | p. 292 |
3.2 Computing the Distance | p. 293 |
4 Correlation Kernels for Non-stationary Time Series | p. 294 |
5 Invariance Via Canonization | p. 295 |
6 Discussion | p. 297 |
References | p. 298 |
Stochastic Realization for Stochastic Control with Partial Observations | p. 301 |
1 Introduction | p. 301 |
2 Problem Formulation | p. 302 |
3 The Classical Approach | p. 303 |
4 The Stochastic Realization Approach to Stochastic Control with Partial Observations | p. 305 |
5 Special Cases | p. 306 |
6 Concluding Remarks | p. 312 |
References | p. 312 |
Experiences from Subspace System Identification - Comments from Process Industry Users and Researchers | p. 315 |
1 Introduction | p. 315 |
2 Questions and Answers from the User | p. 317 |
3 Comments from the Researchers | p. 320 |
4 Input Design | p. 321 |
5 Merging Data | p. 322 |
6 Merging of Models | p. 324 |
7 Conclusion | p. 326 |
References | p. 326 |
Recursive Computation of the MPUM | p. 329 |
1 Introduction | p. 329 |
2 Problem Statement | p. 330 |
3 Subspace Identification | p. 333 |
4 State Construction by Past/Future Partition | p. 334 |
5 The Hankel Structure and the Past/Future Partition | p. 336 |
6 The Left Kernel of a Hankel Matrix | p. 338 |
7 Recursive Computation of a Module Basis | p. 339 |
8 Concluding Remarks | p. 341 |
8.1 Subspace ID | p. 341 |
8.2 State Construction by Shift-and-Cut | p. 342 |
8.3 Return to the Data | p. 343 |
8.4 Approximation and Balanced Reduction | p. 343 |
8.5 The Complementary System | p. 343 |
References | p. 344 |
New Development of Digital Signal Processing Via Sampled-Data Control Theory | p. 345 |
1 Foreword | p. 345 |
2 Introduction | p. 345 |
3 The Shannon Paradigm | p. 346 |
3.1 Problems in the Shannon Paradigm | p. 347 |
4 Control Theoretic Formulation | p. 349 |
5 Application to Images | p. 352 |
6 Concluding Remarks and Related Work | p. 354 |
References | p. 355 |