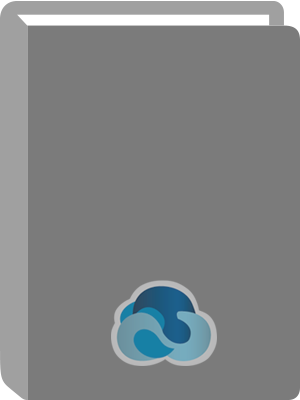
Available:*
Library | Item Barcode | Call Number | Material Type | Item Category 1 | Status |
---|---|---|---|---|---|
Searching... | 30000010242500 | HD61 Q36 2012 | Open Access Book | Book | Searching... |
Searching... | 30000010302422 | HD61 Q36 2012 | Open Access Book | Book | Searching... |
On Order
Summary
Summary
Using real-life examples from the banking and insurance industries, Quantitative Operational Risk Models details how internal data can be improved based on external information of various kinds. Using a simple and intuitive methodology based on classical transformation methods, the book includes real-life examples of the combination of internal data and external information.
A guideline for practitioners, the book begins with the basics of managing operational risk data to more sophisticated and recent tools needed to quantify the capital requirements imposed by operational risk. The book then covers statistical theory prerequisites, and explains how to implement the new density estimation methods for analyzing the loss distribution in operational risk for banks and insurance companies. In addition, it provides:
Simple, intuitive, and general methods to improve on internal operational risk assessment Univariate event loss severity distributions analyzed using semiparametric models Methods for the introduction of underreporting information A practical method to combine internal and external operational risk data, including guided examples in SAS and RMeasuring operational risk requires the knowledge of the quantitative tools and the comprehension of insurance activities in a very broad sense, both technical and commercial. Presenting a nonparametric approach to modeling operational risk data, Quantitative Operational Risk Models offers a practical perspective that combines statistical analysis and management orientations.
Author Notes
Catalina Bolance has been Associate Professor of Quantitative Methods for Economics and Management Science of the Department of Econometrics at University of Barcelona since 2001. She received a PhD in Economics, an M.A. in Marketing, and a B.Sc. in Statistics at the University of Barcelona. She is currently member of the research group Risk in Finance and Insurance and is a specialist in applied nonparametric methods. She has coauthored several undergraduate books on applied statistics that are widely used in Spanish universities and has also published in high-quality scientific journals like Insurance: Mathematics and Economics, Statistics, and Astin Bulletin--The Journal of the International Actuarial Association, the journal of the International Actuarial Association. She has supervised many Master''s and Ph.D. theses with an outstanding tutoring record. Since 2010 she has participated in a project of the London School of Economics on long-term care insurance sponsored by the AXA research fund. In 2004 she received the insurance international prize awarded by MAPFRE.
Montserrat Guillen has been Chair Professor of Quantitative Methods at the University of Barcelona since 2001 and director of the research group on Risk in Finance and Insurance. She received an M.S. degree in Mathematics and Mathematical Statistics in 1987, and a Ph.D. degree in Economics from the University of Barcelona in 1992. She also received theM.A. degree in Data Analysis from the University of Essex, United Kingdom. She was Visiting Research faculty at the University of Texas at Austin (USA) in 1994. She holds a visiting professor position at the University of Paris II, where she teaches Insurance Econometrics. Her research focuses on actuarial statistics and quantitative risk management. Since 2005 she has been an associate editor for the Journal of Risk and Insurance, the official journal of the American Risk and Insurance Association, a senior editor of Astin Bulletin--The Journal of the International Actuarial Association, the journal of the International Actuarial Association since 2009, and chief editor of SORT--Statistics and Operations Research Transactions since 2006. She was elected Vice President of the European Group of Risk and Insurance Economists at the World Congress of Risk and Insurance Economics in 2010.
Jim Gustafsson has been Head of Nordic Quantitative Advisory Services at Ernst & Young since 2011. He has 10 years of business experience in the insurance and consultancy sector, where he was an employee for several years in the insurance Group RSA with positions such as Actuary, Operational Risk Specialist, Head of Research, and Enterprise Risk Control Director. He received a B.Sc. in Mathematical Statistics in 2004, an M.Sc. in Mathematics in 2005 from Lund University, and a Ph.D. degree in Actuarial Science from the University of Copenhagen in 2009. He is author of over a dozen published articles on actuarial statistics and operational risk quantification, and was awarded for the "Best academic Paper" by the Operational Risk and Compliance Magazine in 2007. He was recognized as a "Top 50" Face of Operational Risk by Operational Risk and ComplianceMagazine in 2009; this award acknowledges the contribution that the recipient has made, and continues to make, to the discipline of operational risk. He is a sought-after speaker in Risk Management and Actuarial conferences and a member of the Editorial Board for the international journal Insurance Markets and Companies: Analyses and Actuarial Computations.
Jens Perch Nielsen has been Professor of Actuarial Statistics at Cass Business School in London and CEO of the Denmark based knowledge company Festina Lente. He has a history of combining high academic standards with the immediate practical needs of the insurance industry. Through his company he has managed projects on operational risk, reserving, capital allocation, and risk-adjusted cross-selling methods in non-life-insurance, and he has academic publications in all these areas. In life and pension insurance he has conducted professional work on product development, asset allocation, longevity models and econometric projections and on bread and butter type actuarial day-to-day work. His Ph.D. from UC-Berkeley was in Biostatistics and he is still working on his original topic of general nonparametric smoothing techniques in regression, density and hazard estimation.
Table of Contents
1 Understanding Operational Risk | p. 1 |
1.1 Introduction | p. 1 |
1.2 Our Approach to Operational Risk Quantification | p. 2 |
1.3 Regulatory Framework | p. 3 |
1.4 The Fundamentals of Calculating Operational Risk Capital | p. 5 |
1.5 Notation and Definitions | p. 6 |
1.6 The Calculation of Operational Risk Capital in Practice | p. 8 |
1.7 Organization of the Book | p. 10 |
1.8 Further Reading and Bibliographic Notes | p. 11 |
2 Operational Risk Data and Parametric Models | p. 13 |
2.1 Introduction | p. 13 |
2.2 Internal Data and External Data | p. 14 |
2.3 Basic Parametric Severity Distributions | p. 16 |
2.4 The Generalized Champernowne Distribution | p. 19 |
2.5 Quantile Estimation | p. 24 |
2.6 Further Reading and Bibliographic Notes | p. 25 |
3 Semiparametric Models for Operational Risk Severities | p. 29 |
3.1 Introduction | p. 29 |
3.2 Classical Kernel Density Estimation | p. 30 |
3.3 Transformation Method | p. 33 |
3.3.1 Asymptotic Theory for the Transformation Kernel Density Estimator | p. 35 |
3.3.2 Transformation Method with a Cumulative Distribution Function | p. 36 |
3.4 Bandwidth Selection | p. 38 |
3.5 Boundary Correction | p. 39 |
3.6 Transformation with the Generalized Champernowne Distribution | p. 41 |
3.7 Results for the Operational Risk Data | p. 43 |
3.8 Further Reading and Bibliographic Notes | p. 44 |
4 Combining Operational Risk Data Sources | p. 49 |
4.1 Why Mixing? | p. 49 |
4.2 Combining Data Sources with the Transformation Method | p. 50 |
4.3 The Mixing Transformation Technique | p. 51 |
4.4 Data Study | p. 52 |
4.5 Further Reading and Bibliographic Notes | p. 55 |
5 Underreporting | p. 57 |
5.1 Introduction | p. 57 |
5.2 The Underreporting Function | p. 58 |
5.3 Publicly Reported Loss Data | p. 60 |
5.4 A Semiparametric Approach to Correction for Underreporting | p. 62 |
5.4.1 Setting up a Model for the Sampling of Operational Risk Claims with Underreporting | p. 63 |
5.4.2 A Transformation Approach to Tail Flattening Accounting for Underreporting | p. 63 |
5.5 An Application to Evaluate Operational Risk with Correction for Underreporting in Publicly Reported Losses | p. 65 |
5.6 An Application to Evaluate Internal Operational Risk with Correction for Underreporting | p. 68 |
5.6.1 Aggregated Analysis Incorporating six Event Risk Categories | p. 70 |
5.6.2 Results | p. 70 |
5.7 Further Reading and Bibliographic Notes | p. 72 |
6 Combining Underreported Internal and External Data for Operational Risk Measurement | p. 73 |
6.1 Introduction | p. 73 |
6.2 Data Availability | p. 74 |
6.2.1 Minimum Collection Threshold | p. 75 |
6.2.2 Modeling Each Data Set Separately | p. 76 |
6.3 Underreporting Losses | p. 77 |
6.3.1 Correcting for Underreporting and Collection Thresh-old | p. 79 |
6.3.2 Including Underreporting in the Estimation Procedure | p. 80 |
6.4 A Mixing Model in a Truncation Framework | p. 83 |
6.5 Operational Risk Application | p. 86 |
6.6 Further Reading and Bibliographic Notes | p. 92 |
7 A Guided Practical Example | p. 95 |
7.1 Introduction | p. 95 |
7.2 Descriptive Statistics and Basic Procedures in SAS© | p. 95 |
7.2.1 Fitting the Generalized Champernowne Distribution in SAS© | p. 104 |
7.2.2 Quantile Estimation for Basic Parametric Distributions | p. 108 |
7.2.3 Quantile Estimation for the Generalized Champernowne Distribution | p. 112 |
7.3 Transformation Kernel Estimation | p. 116 |
7.4 Combining Internal and External Data | p. 123 |
7.5 Underreporting Implementation | p. 145 |
7.6 Programming in R | p. 171 |
7.6.1 Basic Functions | p. 171 |
7.6.2 Figures in Chapters 2 and 3 | p. 177 |
Bibliography | p. 203 |
Index | p. 209 |