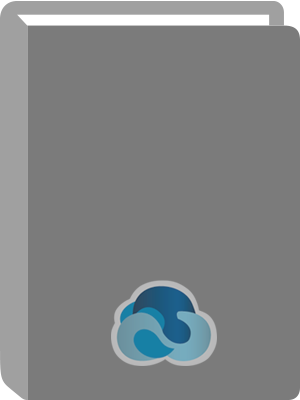
Available:*
Library | Item Barcode | Call Number | Material Type | Item Category 1 | Status |
---|---|---|---|---|---|
Searching... | 30000010128760 | HF5415.2 R67 2005 | Open Access Book | Book | Searching... |
On Order
Summary
Summary
The past decade has seen a dramatic increase in the use of Bayesian methods in marketing due, in part, to computational and modelling breakthroughs, making its implementation ideal for many marketing problems. Bayesian analyses can now be conducted over a wide range of marketing problems, from new product introduction to pricing, and with a wide variety of different data sources.
Bayesian Statistics and Marketing describes the basic advantages of the Bayesian approach, detailing the nature of the computational revolution. Examples contained include household and consumer panel data on product purchases and survey data, demand models based on micro-economic theory and random effect models used to pool data among respondents. The book also discusses the theory and practical use of MCMC methods.
Written by the leading experts in the field, this unique book:
Presents a unified treatment of Bayesian methods in marketing, with common notation and algorithms for estimating the models. Provides a self-contained introduction to Bayesian methods. Includes case studies drawn from the authors' recent research to illustrate how Bayesian methods can be extended to apply to many important marketing problems. Is accompanied by an R package, bayesm , which implements all of the models and methods in the book and includes many datasets. In addition the book's website hosts datasets and R code for the case studies. Bayesian Statistics and Marketing provides a platform for researchers in marketing to analyse their data with state-of-the-art methods and develop new models of consumer behaviour. It provides a unified reference for cutting-edge marketing researchers, as well as an invaluable guide to this growing area for both graduate students and professors, alike.Author Notes
Peter E. Rossi is James Collins Professor of Marketing, Statistics and Economics at UCLA Anderson School of Management. Greg M. Allenby is the author of Bayesian Statistics and Marketing, published by Wiley.
Table of Contents
1 Introduction |
1.1 A Basic Paradigm for Marketing Problems |
1.2 A Simple Example |
1.3 Benefits and Costs of the Bayesian Approach |
1.4 An Overview of Methodological Material and Case Studies |
1.5 Computing and This Book |
Acknowledgements |
2 Bayesian Essentials |
2.0 Essential Concepts from Distribution Theory |
2.1 The Goal of Inference and Bayes' Theorem |
2.2 Conditioning and the Likelihood Principle |
2.3 Prediction and Bayes |
2.4 Summarizing the Posterior |
2.5 Decision Theory, Risk, and the Sampling Properties of Bayes Estimators |
2.6 Identification and Bayesian Inference |
2.7 Conjugacy, Sufficiency, and Exponential Families |
2.8 Regression and Multivariate Analysis Examples |
2.9 Integration and Asymptotic Methods |
2.10 Importance Sampling |
2.11 Simulation Primer for Bayesian Problems |
2.12 Simulation from Posterior of Multivariate Regression Model |
3 Markov Chain Monte Carlo Methods |
3.1 Markov Chain Monte Carlo Methods |
3.2 A Simple Example: Bivariate Normal Gibbs Sampler |
3.3 Some Markov Chain Theory |
3.4 Gibbs Sampler |
3.5 Gibbs Sampler for the Seemingly Unrelated Regression Model |
3.6 Conditional Distributions and Directed Graphs |
3.7 Hierarchical Linear Models |
3.8 Data Augmentation and a Probit Example |
3.9 Mixtures of Normals |
3.10 Metropolis Algorithms |
3.11 Metropolis Algorithms Illustrated with the Multinomial Logit Model |
3.12 Hybrid Markov Chain Monte Carlo Methods |
3.13 Diagnostics |
4 Unit-Level Models and Discrete Demand |
4.1 Latent Variable Models |
4.2 Multinomial Probit Model |
4.3 Multivariate Probit Model |
4.4 Demand Theory and Models Involving Discrete Choice |
5 Hierarchical Models for Heterogeneous Units |
5.1 Heterogeneity and Priors |
5.2 Hierarchical Models |
5.3 Inference for Hierarchical Models |
5.4 A Hierarchical Multinomial Logit Example |
5.5 Using Mixtures of Normals |
5.6 Further Elaborations of the Normal Model of Heterogeneity |
5.7 Diagnostic Checks of the First-Stage Prior |
5.8 Findings and Influence on Marketing Practice |
6 Model Choice and Decision Theory |
6.1 Model Selection |
6.2 Bayes Factors in the Conjugate Setting |
6.3 Asymptotic Methods for Computing Bayes Factors |
6.4 Computing Bayes Factors Using Importance Sampling |
6.5 Bayes Factors Using MCMC Draws |
6.6 Bridge Sampling Methods |
6.7 Posterior Model Probabilities with Unidentified Parameters |
6.8 Chib's Method |
6.9 An Example of Bayes Factor Computation: Diagonal Multinomial Probit Models |
6.10 Marketing Decisions and Bayesian Decision Theory |
6.11 An Example of Bayesian Decision Theory: Valuing Household Purchase Information |
7 Simultaneity |
7.1 A Bayesian Approach to Instrumental Variables |
7.2 Structural Models and Endogeneity/Simultaneity |
7.3 Nonrandom Marketing Mix Variables |
Case Study 1: A Choice Model for Packaged Goods: Dealing with Discrete Quantities and Quantity Discounts |
Background |
Model |
Data |
Results |
Discussion |
R Implementation |
Case Study 2: Modeling Interdependent Consumer Preferences |
Background |
Model |
Data |
Results |
Discussion |
R Implementation |
Case Study 3: Overcoming Scale Usage Heterogeneity |
Background |
Model |
Priors and MCMC Algorithm |
Data |
Discussion |
R Implementation |
Case Study 4: A Choice Model with Conjunctive Screening Rules |
Background |
Model |
Data |
Results |
Discussion |
R Implementation |
Case Study 5: Modeling Consumer Demand for Variety |
Background |
Model |
Data |
Results |
Discussion |
R Implementation |
Appendix A An Introduction to Hierarchical Bayes Modeling in R |
A.1 Setting Up the R Environment |