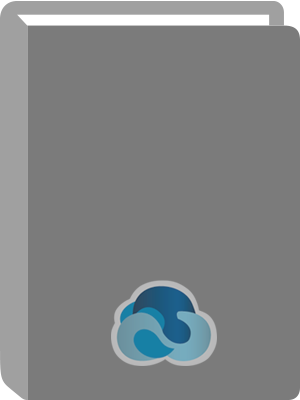
Available:*
Library | Item Barcode | Call Number | Material Type | Item Category 1 | Status |
---|---|---|---|---|---|
Searching... | 30000010219150 | QP702.G577 A64 2009 | Open Access Book | Book | Searching... |
On Order
Summary
Summary
A Focused, State-of-the-Art Overview of This Evolving Field
Presents Various Techniques for Glycoinformatics
The development and use of informatics tools and databases for glycobiology and glycomics research have increased considerably in recent years. In addition to accumulating well-structured glyco-related data, researchers have now developed semi-automated methods for the annotation of mass spectral data and algorithms for capturing patterns in glycan structure data. These techniques have enabled researchers to gain a better understanding of how these complex structures affect protein function and other biological processes, including cancer.
One of the few up-to-date books available in this important area, Glycome Informatics: Methods and Applications covers all known informatics methods pertaining to the study of glycans. It discusses the current status of carbohydrate databases, the latest analytical techniques, and the informatics needed for rapid progress in glycomics research.
Providing an overall understanding of glycobiology, this self-contained guide focuses on the development of glycome informatics methods and current problems faced by researchers. It explains how to implement informatics methods in glycobiology. The author includes the required background material on glycobiology as well as the mathematical concepts needed to understand advanced mining and algorithmic techniques. She also suggests project themes for readers looking to begin research in the field.
Author Notes
Kiyoko F. Aoki-Kinoshita simultaneously received her bachelor's and master'sdegrees of science in computer science from Northwestern University in 1996, after which she received her doctorate in computer engineering from Northwestern in 1999 under Dr. D. T. Lee. She was employed at BioDiscovery, Inc. in Los Angeles, California as a senior software engineer before moving to Kyoto, Japan, to work as a post-doctoral researcher at the Bioinformatics Center, Institute of Chemical Research, Kyoto University, under Drs. Hiroshi Mamitsuka and Minoru Kanehisa. There, she developed various algorithmic and data mining methods for analyzing the glycan structure data that were accumulated in the KEGG GLYCAN database. Since then, she has joined the faculty in the Department of Bioinformatics, Faculty of Engineering, Soka University, in Tokyo, Japan and is now an associate professor teaching bioinformatics. She is also involved in several research projects pertaining to the understanding of glycan function based on their structure as well as the recognition patterns of glycan structures by other proteins and even viruses. She has also begun developing a Web resource called RINGS (Resource for INformatics of Glycomes at Soka) that is still in its infancy, but is intended to freely provide many of the informatics algorithms and methods described in this book over the Web such that scientists may utilize them easily.
Table of Contents
List of Tables | p. xi |
List of Figures | p. xiii |
About the Author | p. xvii |
1 Introduction to Glycobiology | p. 1 |
1.1 Roles of carbohydrates | p. 1 |
1.2 Glycan structures | p. 2 |
1.3 Glycan classes | p. 6 |
1.4 Glycan biosynthesis | p. 13 |
1.4.1 N-linked glycans | p. 13 |
1.4.2 O-linked glycans | p. 16 |
1.4.3 Glycosaminoglycans (GAGs) | p. 16 |
1.4.4 Glycosphingolipids (GSLs) | p. 17 |
1.4.5 GPI anchors | p. 19 |
1.4.6 LPS | p. 19 |
1.5 Glycan motifs | p. 20 |
1.6 Potential for drug discovery | p. 22 |
2 Background | p. 25 |
2.1 Glycan nomenclature | p. 25 |
2.1.1 InChIÖ | p. 25 |
2.1.2 (Extended) IUPAC format | p. 27 |
2.1.3 CarbBank format | p. 30 |
2.1.4 KCF format | p. 31 |
2.1.5 LINUCS format | p. 32 |
2.1.6 BCSDB format | p. 34 |
2.1.7 Linear Code" | p. 37 |
2.1.8 GlycoCT format | p. 40 |
2.1.9 XML representations | p. 46 |
2.2 Lectin-glycan interactions | p. 48 |
2.2.1 Families and types of lectins | p. 50 |
2.2.2 Carbohydrate-binding mechanism of lectins | p. 57 |
2.3 Carbohydrate-carbohydrate interactions | p. 58 |
3 Databases | p. 61 |
3.1 Glycan structure databases | p. 61 |
3.1.1 KEGG GLYCAN | p. 62 |
3.1.2 GLYCOSCIENCES.de | p. 68 |
3.1.3 CFG | p. 74 |
3.1.4 BCSDB | p. 82 |
3.1.5 GLYCO3D | p. 85 |
3.1.6 MonoSaccharideDB | p. 86 |
3.1.7 GlycomeDB | p. 89 |
3.2 Glyco-gene databases | p. 90 |
3.2.1 KEGG BRITE | p. 91 |
3.2.2 CFG | p. 91 |
3.2.3 GGDB | p. 94 |
3.2.4 CAZy | p. 94 |
3.3 Lipid databases | p. 96 |
3.3.1 SphingoMAP© | p. 96 |
3.3.2 LipidBank | p. 97 |
3.3.3 LMSD | p. 98 |
3.4 Lectin databases | p. 101 |
3.4.1 Lectines | p. 101 |
3.4.2 Animal Lectin DB | p. 101 |
3.5 Others | p. 101 |
3.5.1 GlycoEpitopeDB | p. 101 |
3.5.2 ECODAB | p. 102 |
3.5.3 SugarBindDB | p. 106 |
4 Glycome Informatics | p. 107 |
4.1 Terminology and notations | p. 107 |
4.2 Algorithmic techniques | p. 108 |
4.2.1 Tree structure alignment | p. 108 |
4.2.2 Linkage analysis using score matrices | p. 110 |
4.2.3 Glycan variation map | p. 112 |
4.3 Bioinformatic methods | p. 114 |
4.3.1 Glycan structure prediction from glycogene microarrays | p. 114 |
4.3.2 Glyco-gene sequence and structure analysis | p. 116 |
4.3.3 Glyco-related pathway analysis | p. 119 |
4.3.4 Mass spectral data annotation | p. 124 |
4.4 Data mining techniques | p. 130 |
4.4.1 Kernel methods | p. 131 |
4.4.2 Frequent subtree mining | p. 138 |
4.4.3 Probabilistic models | p. 142 |
4.5 Glycomics tools | p. 173 |
4.5.1 Visualization tools | p. 173 |
4.5.2 Pathway analysis tools | p. 177 |
4.5.3 PDB data analysis | p. 178 |
4.5.4 3D analysis tools | p. 179 |
4.5.5 Molecular dynamics | p. 182 |
4.5.6 Spectroscopic tools | p. 186 |
4.5.7 NMR tools | p. 189 |
5 Potential Research Projects | p. 193 |
5.1 Sequence and structural analyses | p. 193 |
5.1.1 Glycan score matrix | p. 194 |
5.1.2 Visualization | p. 194 |
5.2 Databases and techniques to integrate heterogeneous data sets | p. 195 |
5.3 Automated characterization of glycans from MS data | p. 196 |
5.4 Prediction of glycans from data other than MS | p. 196 |
5.5 Biomarker prediction | p. 197 |
5.6 Systems analyses | p. 197 |
5.7 Drug discovery | p. 198 |
A Sequence Analysis Methods | p. 199 |
A.1 Pairwise sequence alignment (dynamic programming) | p. 199 |
A.1.1 Dynamic programming | p. 199 |
A.1.2 Sequence alignment | p. 202 |
A.2 BLOSUM (BLOcks Substitution Matrix) | p. 205 |
B Machine Learning Methods | p. 207 |
B.1 Kernel methods and SVMs | p. 207 |
B.2 Hidden Markov models | p. 211 |
B.2.1 The three problems of interest for HMMs | p. 213 |
B.2.2 Expectation-Maximization (EM) algorithm | p. 215 |
B.2.3 Hidden tree Markov models | p. 216 |
B.2.4 Profile Hidden Markov models (profile HMMs) | p. 218 |
C Glycomics Technologies | p. 221 |
C.1 Mass spectrometry (MS) | p. 221 |
C.1.l MALDI-MS | p. 222 |
C.1.2 FT-ICR | p. 223 |
C.1.3 LC-MS (HPLC) | p. 224 |
C.1.4 Tandem MS | p. 224 |
C.2 Nuclear magnetic resonance (NMR) | p. 225 |
References | p. 227 |
Index | p. 241 |