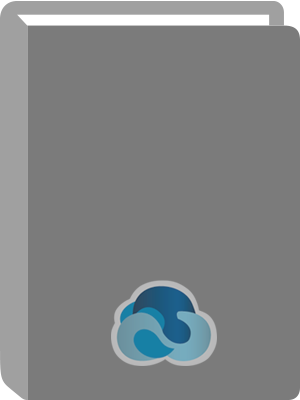
Available:*
Library | Item Barcode | Call Number | Material Type | Item Category 1 | Status |
---|---|---|---|---|---|
Searching... | 30000010112860 | TA1637 V37 2004 | Open Access Book | Book | Searching... |
Searching... | 30000010112857 | TA1637 V37 2004 | Open Access Book | Book | Searching... |
Searching... | 30000010112858 | TA1637 V37 2004 | Open Access Book | Book | Searching... |
Searching... | 30000010112859 | TA1637 V37 2004 | Open Access Book | Book | Searching... |
On Order
Summary
Summary
Over the last fifty years, a large number of spaceborne and airborne sensors have been employed to gather information regarding the earth's surface and environment. As sensor technology continues to advance, remote sensing data with improved temporal, spectral, and spatial resolution is becoming more readily available. This widespread availability of enormous amounts of data has necessitated the development of efficient data processing techniques for a wide variety of applications. In particular, great strides have been made in the development of digital image processing techniques for remote sensing data. The goal has been efficient handling of vast amounts of data, fusion of data from diverse sensors, classification for image interpretation, and development of user-friendly products that allow rich visualization. This book presents some new algorithms that have been developed for high dimensional datasets, such as multispectral and hyperspectral imagery. The contents of the book are based primarily on research carried out by some members and alumni of the Sensor Fusion Laboratory at Syracuse University.
Table of Contents
Hyperspectral Sensors and Applications |
Overview of Image Processing |
Mutual Information: A Similarity Measure for Intensity Based Image Registration |
Independent Component Analysis |
Support Vector Machines |
Markov Random Field Models |
Applications: MI Based Registration of Multi-Sensor and Multi-Temporal Images |
Feature Extraction from Hyperspectral Data Using ICA |
Hyperspectral Classification using ICA Based Mixture Model |
Support Vector Machines for Classification of Multi- and Hyperspectral Data |
An MRF Model Based Approach for Sub-pixel Mapping from Hyperspectral Data |
Image Change Detection and Fusion Using MRF Models |