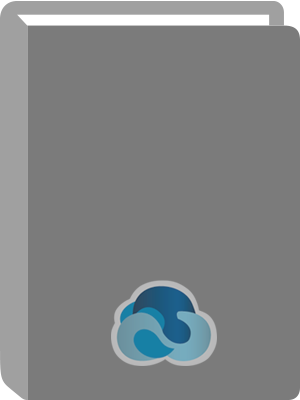
Available:*
Library | Item Barcode | Call Number | Material Type | Item Category 1 | Status |
---|---|---|---|---|---|
Searching... | 30000010229664 | QA278.8 M45 2009 | Open Access Book | Book | Searching... |
On Order
Summary
Summary
Deconvolution problems occur in many ?elds of nonparametric statistics, for example, density estimation based on contaminated data, nonparametric - gression with errors-in-variables, image and signal deblurring. During the last two decades, those topics have received more and more attention. As appli- tions of deconvolution procedures concern many real-life problems in eco- metrics, biometrics, medical statistics, image reconstruction, one can realize an increasing number of applied statisticians who are interested in nonpa- metric deconvolution methods; on the other hand, some deep results from Fourier analysis, functional analysis, and probability theory are required to understand the construction of deconvolution techniques and their properties so that deconvolution is also particularly challenging for mathematicians. Thegeneraldeconvolutionprobleminstatisticscanbedescribedasfollows: Our goal is estimating a function f while any empirical access is restricted to some quantity h = f?G = f(x?y)dG(y), (1. 1) that is, the convolution of f and some probability distribution G. Therefore, f can be estimated from some observations only indirectly. The strategy is ^ estimating h ?rst; this means producing an empirical version h of h and, then, ^ applying a deconvolution procedure to h to estimate f. In the mathematical context, we have to invert the convolution operator with G where some reg- ^ ularization is required to guarantee that h is contained in the invertibility ^ domain of the convolution operator. The estimator h has to be chosen with respect to the speci?c statistical experiment.
Table of Contents
1 Introduction | p. 1 |
2 Density Deconvolution | p. 5 |
2.1 Additive Measurement Error Model | p. 5 |
2.2 Estimation Procedures | p. 9 |
2.2.1 Kernel Methods | p. 10 |
2.2.2 Wavelet-based Methods | p. 14 |
2.2.3 Ridge-Parameter Approach | p. 21 |
2.3 General Consistency | p. 23 |
2.4 Optimal Convergence Rates | p. 32 |
2.4.1 Smoothness Classes/Types of Error Densities | p. 33 |
2.4.2 Mean Squared Error: Upper Bounds | p. 36 |
2.4.3 Mean Integrated Squared Error: Upper Bounds | p. 41 |
2.4.4 Asymptotic Normality | p. 46 |
2.4.5 Mean Squared Error: Lower Bounds | p. 50 |
2.4.6 Mean Integrated Squared Error: Lower Bounds | p. 58 |
2.5 Adaptive Bandwidth Selection | p. 63 |
2.5.1 Cross Validation | p. 65 |
2.6 Unknown Error Density | p. 78 |
2.6.1 Deterministic Constraints | p. 80 |
2.6.2 Additional Data | p. 85 |
2.6.3 Replicated Measurements | p. 88 |
2.7 Special Problems | p. 92 |
2.7.1 Heteroscedastic Contamination | p. 92 |
2.7.2 Distribution and Derivative Estimation | p. 95 |
2.7.3 Other Related Topics | p. 103 |
3 Nonparametric Regression with Errors-in-Variables | p. 107 |
3.1 Errors-in-Variables Problems | p. 107 |
3.2 Kernel Methods | p. 111 |
3.3 Asymptotic Properties | p. 113 |
3.3.1 Consistency | p. 113 |
3.3.2 Optimal Convergence Rates | p. 118 |
3.4 Berkson Regression | p. 133 |
3.4.1 Discrete-Transform Approach | p. 134 |
3.4.2 Convergence Rates | p. 138 |
4 Image and Signal Reconstruction | p. 151 |
4.1 Discrete Observation Scheme and Blind Deconvolution | p. 151 |
4.2 White Noise Model | p. 161 |
4.3 Circular Model and Boxcar Deconvolution | p. 168 |
A Tools from Fourier Analysis | p. 179 |
A.1 Fourier Transforms of L1(R)-Functions | p. 179 |
A.2 Fourier Transforms of L2(R)-Functions | p. 182 |
A.3 Fourier Series | p. 189 |
A.4 Multivariate Case | p. 197 |
B List of Symbols | p. 201 |
References | p. 205 |