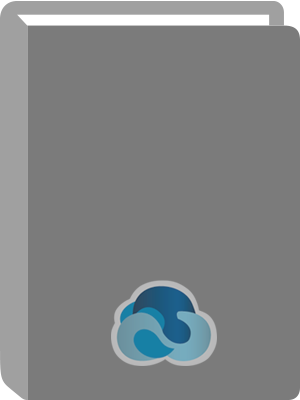
Available:*
Library | Item Barcode | Call Number | Material Type | Item Category 1 | Status |
---|---|---|---|---|---|
Searching... | 30000010343153 | Q325.5 C665 2014 | Open Access Book | Book | Searching... |
Searching... | 33000000016460 | Q325.5 C665 2014 | Open Access Book | Book | Searching... |
On Order
Summary
Summary
The conformal predictions framework is a recent development in machine learning that can associate a reliable measure of confidence with a prediction in any real-world pattern recognition application, including risk-sensitive applications such as medical diagnosis, face recognition, and financial risk prediction. Conformal Predictions for Reliable Machine Learning: Theory, Adaptations and Applications captures the basic theory of the framework, demonstrates how to apply it to real-world problems, and presents several adaptations, including active learning, change detection, and anomaly detection. As practitioners and researchers around the world apply and adapt the framework, this edited volume brings together these bodies of work, providing a springboard for further research as well as a handbook for application in real-world problems.
Author Notes
Vineeth N Balasubramanian is an Assistant Professor in the Department of Computer Science and Engineering at the Indian Institute of Technology, Hyderabad, India. Until July 2013, he was an Assistant Research Professor at the Center for Cognitive Ubiquitous Computing (CUbiC) at Arizona State University (ASU). He holds dual Masters degrees in Mathematics (2001) and Computer Science (2003) from Sri Sathya Sai University, India, and worked at Oracle Corporation for two years until 2005. His PhD dissertation (2010) on the Conformal Predictions framework was nominated for the Outstanding PhD Dissertation at the Department of Computer Science at ASU, as well as for the annual ACM Doctoral Dissertation Award. He was also a recipient of the Gold Medals for Academic Excellence for his performances in the Bachelors program in Math in 1999, and for his Masters program in Computer Science in 2003. His research interests include pattern recognition, machine learning, computer vision and multimedia computing within assistive and healthcare applications. His current research includes extending the Conformal Predictions framework to real-world problem contexts, and newer machine learning problems such as active learning and transfer learning.
Shen-Shyang Ho is an Assistant Professor in the School of Computer Engineering at the Nanyang Technological University (NTU), Singapore. Before joining NTU in January 2012, he was an assistant research scientist at the University of Maryland Institute for Advanced Computer Studies (UMIACS). He received the BS degree in mathematics and computational science from the National University of Singapore in 1999, and the MS and PhD degrees in computer science from George Mason University in 2003 and 2007, respectively. He was formerly a NASA Postdoctoral Program (NPP) fellow affiliated to the Jet Propulsion Laboratory (JPL) and a postdoctoral scholar affiliated to the California Institute of Technology working in the Climate, Oceans, and Solid Earth Science section in the science division at JPL. His research interests include learning from data streams/sequences, adaptive learning, pattern recognition, data mining in spatio-temporal domain and moving objects databases, and machine learning/data mining on mobile devices.
Vladimir Vovk is Professor of Computer Science at Royal Holloway, University of London; he also heads the Computer Learning Research Centre. His research interests include machine learning; predictive and Kolmogorov complexity, randomness, and information; the foundations of probability and statistics. He has published numerous research papers in these fields and two books: "Probability and finance: It's only a game" (with Glenn Shafer, Wiley, New York, 2001; Japanese translation: Iwanami Shoten, Tokyo, 2006) and "Algorithmic learning in a random world" (with Alex Gammerman and Glenn Shafer, Springer, New York, 2005), which is a comprehensive book on the Conformal Predictions framework.
Table of Contents
Section I Theory |
1 The Basic Conformal Prediction Framework |
2 Beyond the Basic Conformal Prediction Framework |
Section II Adaptations |
3 Active Learning using Conformal Prediction |
4 Anomaly Detection |
5 Online Change Detection by Testing Exchangeability |
6 Feature Selection and Conformal Predictors |
7 Model Selection |
8 Quality Assessment |
9 Other Adaptations |
Section III Applications |
10 Biometrics |
11 Diagnostics and Prognostics by Conformal Predictors |
12 Biomedical Applications using Conformal Predictors |
13 Reliable Network Traffic Classification and Demand Prediction |
14 Other Applications |